Last updated: February 13, 2023
Article
Wildlife Monitoring at Organ Pipe Cactus National Monument, 2021
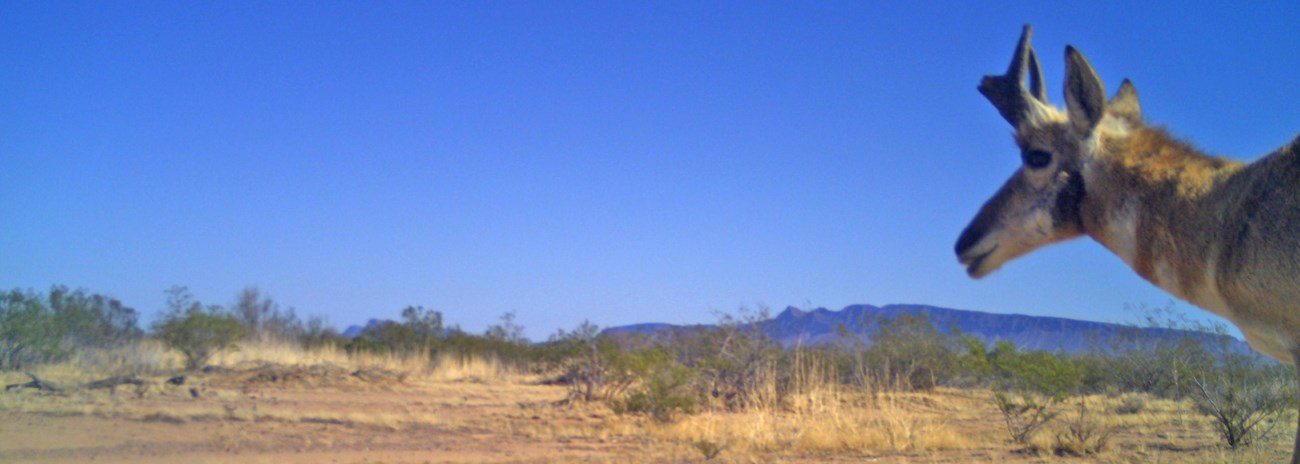
Overview
At National Park Service units across the Sonoran Desert and Apache Highlands, the Sonoran Desert Network (SODN) is monitoring medium- and large-sized mammals using remote wildlife camera traps. The overarching goal of this monitoring, started in 2016, is to detect biologically significant changes in community and population parameters of medium- to large-sized mammals through time in several National Park Service units. The intent is to provide reliable information on the status and trends of mammal populations and communities at various spatial and temporal scales. This is accomplished using passively triggered remote wildlife cameras and a sampling design and methodology that is informative and applicable to addressing park managers’ needs.
Investigating how wildlife occupancy and detection probabilities change through time, and how different factors (including anthropogenic disturbances) impact them, gives us valuable insight into how to best manage and protect species now and into the future. Data collected at Organ Pipe Cactus National Monument (NM) in 2021 (summarized below) are useful on their own to gain species-specific insights, such as new detections within the monument and potential drivers of species distributions. In the future, data from multiple years will be used in occupancy modeling (see Background). We provide an example of an occupancy model for a single-species below and methods for trend analysis are currently in development.
Motivation for wildlife monitoring
The need for wide-scale environmental monitoring to meet conservation needs is increasing. In response to anthropogenic disturbances, such as habitat loss and climate change, many species face pressures that force shifts in their distribution. Expansive tracts of suitable habitat to allow for such shifts are a conservation necessity (Parmesan and Yohe 2003, Thomas et al. 2004). Large protected areas, such as national parks, can provide critical wildlife habitat—but even they are not immune to anthropogenic disturbances (Brashares 2010, Carroll 2010). Knowing how species respond to various pressures requires an understanding of abiotic and multi-species interactions at broad spatial scales. Occupancy modeling of data from remote cameras can help meet this challenge (Post et al. 2009).
Use of cameras
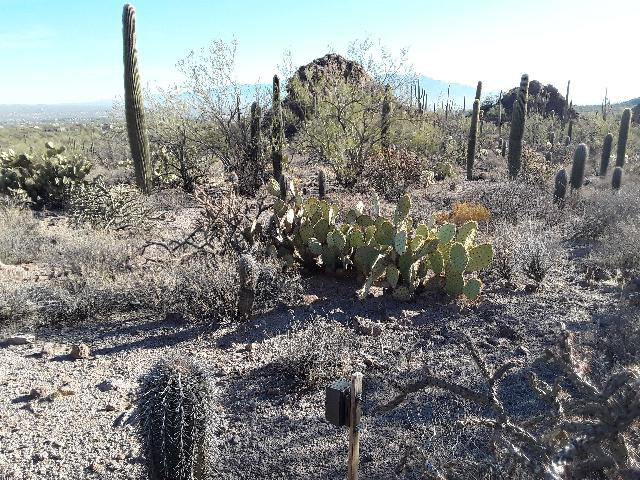
Wildlife camera deployed at Saguaro National Park, Tucson Mountain District.
Remote wildlife cameras are powerful research tools. They are non-invasive and allow researchers to collect data on multiple species simultaneously over large spatial scales. Scientists worldwide have demonstrated the ability of remote cameras to provide robust trend-monitoring data for terrestrial mammals. Remote cameras can capture data on individual animals that are uniquely identifiable, such as jaguars, which enables trend estimations of their broader population through mark-recapture modeling (Steenweg et al. 2015). However, many animals without unique pelage (markings) can only be recognized to the species level, rendering mark-recapture modeling impossible. Instead, occupancy modeling provides a means to monitor distribution (occupancy) trends of numerous species concurrently.
What is occupancy modeling?
In SODN’s monitoring, occupancy is the proportion of an area that is inhabited (occupied) by a species. When a species is detected by a camera, it is classified as present. If a species is not detected by a camera, it may be either truly absent, or present but undetected. This issue of imperfect surveys (i.e., when the probability of detecting a species is less than 1) can be statistically accounted for with occupancy modeling. A rigorous framework for occupancy modeling has existed since 2002 (MacKenzie et al. 2002) and is in strong subsequent development (MacKenzie et al. 2006, 2018). Therefore, we utilize occupancy modeling for the data collected by wildlife cameras at Saguaro National Park’s TMD and other parks.
While the data tell us where a species occurs, they do not tell us why a species does or does not occur at a particular location. We can assess the driving contributors to a species’ distribution by evaluating the effects of covariates on occupancy and detection probability. Some environmental influences, such as elevation, do not change during a sampling window and likely influence occupancy probability. Other environmental variables, such as temperature or precipitation, change day-to-day and may influence detection probability (e.g., species may be harder to see and less active in excessive heat). By including covariates, we can evaluate their influence on wildlife occupancy and detection probability and begin to understand why a species is detected at one place and/or time but not another.
Why is this information useful to park managers?
Long-term monitoring allows us to evaluate trends in parameters of management interest. Modeling occupancy over several years provides SODN and park managers with information on the status and trends of mammal species, including their population distribution and stability. Occupancy is often used as a surrogate for abundance (MacKenzie et al. 2018), so pooling annual occupancy estimates can help us determine how stable or unstable a wildlife population is. When analyzed with occupancy models, SODN’s photographic dataset enables us to monitor trends of numerous terrestrial mammal species, including whether they are potentially increasing, stable, or decreasing.
Depending on the covariates used in the modeling process, we can also plot trends of covariate influence on wildlife occupancy and detection probabilities. For example, assessing the influence of temperature and precipitation on wildlife occupancy and detection is helpful when we want to understand the potential impacts of climate change on species or populations of interest.
Effort
In March 2021, SODN field crews deployed 61 Cuddeback E2 Series wildlife cameras at non-baited, pre-established monitoring locations throughout Organ Pipe Cactus NM. These permanent sampling locations were allocated mostly through a Reversed Randomized Quadrant-Recursive Raster (RRQRR) spatially balanced design (Theobald et al. 2007, Hubbard et al. 2012). Technicians followed standard operating procedures to ensure the cameras were deployed consistently (Hubbard et al. in prep). For example, the cameras were placed in the same locations and employed the same settings as in prior years. The cameras were either mounted on a stake in the ground or strapped to a secure structure, such as a tree.
Relying on infrared and motion-trigger technology, the cameras took a photo whenever they detected motion or a sudden temperature change in their detection zone. They collected data for a sampling period from March 11 until April 25 and were then retrieved. Technicians again followed standard operating procedures to ensure the cameras were retrieved consistently (Hubbard et al. in prep), including documenting each camera’s functionality and properly removing and storing their memory cards.
Back at the office, technicians analyzed the contents of the memory cards. This process begins by downloading the photos and sorting them to remove “false triggers,” which are photos that do not contain an animal. A photo that does contain an animal is classified as a “detection.” From there, the dataset is further sorted using the Colorado Parks and Wildlife Photo Warehouse Database, which helps us categorize photos and attribute spatial and species metadata to them (Ivan and Newkirk 2016). Finally, a metadata export is produced for use in occupancy analysis.
The fieldwork associated with camera deployment and retrieval is typically accomplished with the assistance of citizen scientists. But due to safety concerns related to the COVID-19 pandemic, no citizen scientists participated in this protocol during 2021.
Single-species single-season occupancy models
Many large-scale wildlife occupancy trend models require data from consecutive years, which renders data from a single sampling period inadequate for trend analysis. However, the terrestrial mammal data we collect from a single field season can still be informative and incorporated into different occupancy models that provide information on specific species or covariates of interest.
As an example, we modeled occupancy using a single-season, single-species approach (MacKenzie et al. 2002, 2006) with package “unmarked” in R (Fiske and Chandler 2011). To minimize heterogeneity caused by short periods of high activity in front of the cameras, we grouped our 2021 detection data into seven-day sampling periods (Bowler et al. 2017, MacKenzie et al. 2018, Kays et al. 2020). We then selected nine target species for analysis based on two criteria: rarity and body size. We deemed terrestrial mammal species reliably detectable and identifiable by cameras if they were at least 43 centimeters long, which is equivalent to the size of a rock squirrel (Spermophilus variegatus). Smaller animals can be difficult to identify correctly through photographs, especially nocturnal animals with similar characteristics. For this reason, we excluded smaller rodents, such as chipmunks, rats, and mice from analysis. Furthermore, we only included species for analysis that were detected at least once on >30% of sampled sites to minimize statistical modeling errors. This excluded rarer species, such as Sonoran pronghorn (Antilocapra americana sonoriensis).
Because we had little initial information about which covariates might influence occupancy and detection probabilities, we chose backwards elimination over an information-theoretic approach (Steidl 2006, 2007) to identify covariates with explanatory power (Ramsey and Schafer 2013). We first identified covariates that influenced detection probability with an intercept-only model, then used those in models for occupancy, retaining only those covariates with some explanatory power (p <0.10).
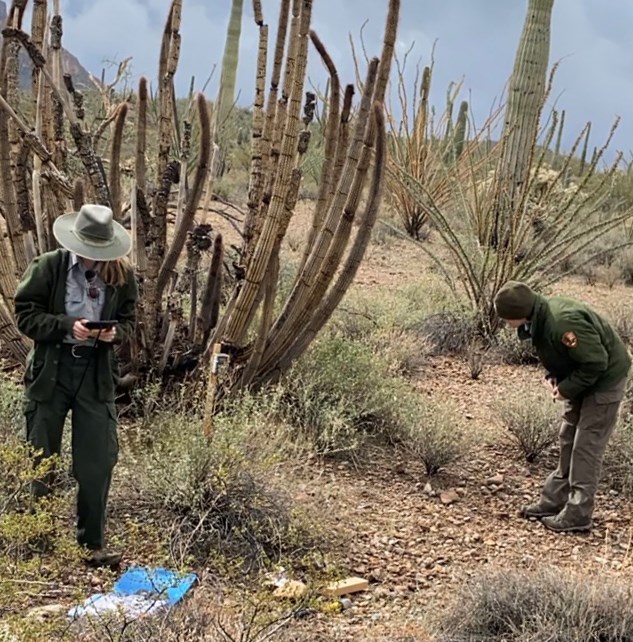
Results for 2021
Detections
In 2021, field crews deployed 61 wildlife cameras at Organ Pipe Cactus National Monument (NM). The cameras have been deployed during the same time period and in the same locations annually since 2016 (except for 2019, due to the federal government shutdown). The cameras collected data from March 11 to April 25, 2021, and were then retrieved. The resulting photos were processed and attributed with metadata, including species, spatial, and temporal information.
The cameras recorded 809 total detections (i.e., animal photographs), including 478 detections of mammals identified to 16 species (see photo gallery and table below) and 272 detections identified to genus or family. Four mammalian families or genera could not be identified at the species level due to insufficient visual evidence. Three bird species were also detected, along with one bird that could not be identified further.
Class | Common name | Scientific name | Number of detections |
---|---|---|---|
Mammal | unknown jackrabbit | Lepus sp. | 198 |
Mammal | black-tailed jackrabbit | Lepus californicus | 115 |
Mammal | mule deer | Odocoileus hemionus | 90 |
Mammal | coyote | Canis latrans | 63 |
Mammal | desert cottontail | Sylvilagus audubonii | 61 |
Mammal | unknown canid | Canidae | 61 |
Mammal | javelina | Pecari tajacu | 52 |
Mammal | unknown mammal | Mammalia | 49 |
Mammal | gray fox | Urocyon cinereoargenteus | 43 |
Mammal | bobcat | Lynx rufus | 16 |
Mammal | unknown fox | Urocyon or Vulpes sp. | 11 |
Mammal | antelope jackrabbit | Lepus alleni | 10 |
Mammal | white-tailed deer | Ococoileus virginianus | 6 |
Mammal | kit fox | Vulpes macrotis | 5 |
Mammal | American badger | Taxidea taxus | 4 |
Mammal | bighorn sheep | Ovis canadensis | 4 |
Mammal | rock squirrel | Spermophilus variegatus | 3 |
Mammal | Sonoran pronghorn | Antilocapra americana sonoriensis | 3 |
Mammal | Harris's antelope squirrel | Ammospermophilus harrisii | 2 |
Mammal | unknown deer | Odocoileus sp. | 2 |
Mammal | western spotted skunk | Spilogale gracilis | 1 |
Total mammals | -- | -- | 799 |
Bird | Gambel's quail | Callipepla gambelii | 4 |
Bird | common raven | Corvus corax | 3 |
Bird | unknown bird | Aves | 2 |
Bird | red-tailed hawk | Buteo jamaicensis | 1 |
Total non-mammals | -- | -- | 10 |
Total | -- | -- | 809 |
At 24 camera locations, at least one species was detected that had not been previously detected at those sites, indicating an increase in wildlife activity at site-specific locations. Notable detections included American badger (Taxidea taxus), antelope jackrabbit (Lepus alleni), bighorn sheep (Ovis canadensis), Sonoran pronghorn (Antilocapra americana sonoriensis), western spotted skunk (Spilogale gracilis), and white-tailed deer (Odocoileus virginianus). These species were all detected at new locations during the 2021 sampling window. It is an encouraging sign that these species are active within Organ Pipe Cactus NM, because they are generally uncommon or even rare to observe at the monument.
The number of statistically significant wildlife photos (i.e., photos containing an animal) collected from sampling at Organ Pipe Cactus NM has ranged from 575 to 3,738 annually. We currently have five years of data (2016–2021, excluding 2019 due to the federal government shutdown).
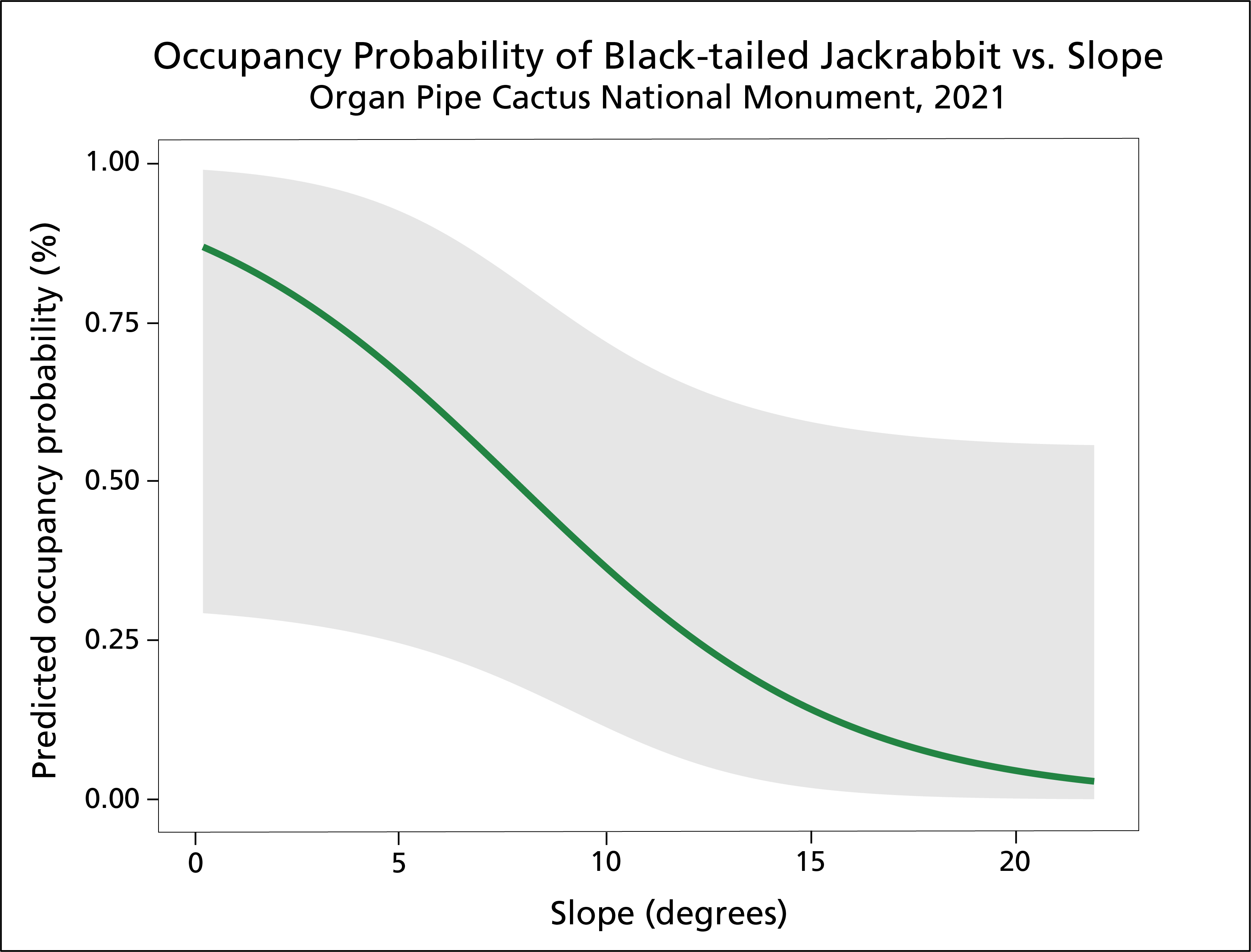
Single-season, single species analysis example
At left is the output for a single-season, single-species occupancy model for black-tailed jackrabbit (Lepus californicus) in Organ Pipe Cactus NM, based on SODN’s terrestrial mammal data collected in 2021. This model illustrates the significant influence of slope on the occupancy probability of black-tailed jackrabbit. More specifically, the model shows occupancy of black-tailed jackrabbit decreasing as slope increases. In the flatter areas of Organ Pipe Cactus NM, occupancy probability of black-tailed jackrabbit is high (60–87% occupied). However, the occupancy probability of black-tailed jackrabbit drops significantly (less than 25%) in steep areas (above 12 degrees incline) within the monument.
The model suggests that black-tailed jackrabbit prefer to inhabit flatter areas over steeper areas in Organ Pipe Cactus NM. This is likely because the flatter areas provide easier access to food, water, and cover compared to the monument’s steeper areas that are more barren, rugged, and harder to traverse. This outcome provides park managers with insight into how black-tailed jackrabbit utilize different areas of the monument. The model also illustrates how data from a single sampling period can provide useful information.
Brashares, J. S. 2010. Filtering wildlife. Science 329:402.
Bowler, M. T., M. W. Tobler, B. A. Endress, M. P. Gilmore, and M. J. Anderson. 2017. Estimating mammalian species richness and occupancy in tropical forest canopies with arboreal camera traps. Remote Sensing in Ecology and Conservation 3:146–157.
Carroll, C. 2010. Role of climatic niche models in focal-species-based conservation planning: Assessing potential effects of climate change on Northern Spotted Owl in the Pacific Northwest, USA. Biological Conservation 143:1432–1437.
Fiske, I., and R. Chandler. 2011. unmarked - an R package for fitting hierarchical models of wildlife occurrence and abundance. Journal of Statistical Software 43:1–23.
Hubbard, J. A., C. L. McIntyre, S. E. Studd, T. Naumann, D. Angell, K. Beaupre, B. Vance, and M. K. Connor. 2012. Terrestrial vegetation and soils monitoring protocol and standard operating procedures: Sonoran Desert and Chihuahuan Desert networks, version 1.1. Natural Resource Report NPS/SODN/NRR—2012/509, National Park Service, Fort Collins, Colorado.
Hubbard, J. A., E. C. Dillingham, and A. Buckisch. In prep. Terrestrial mammal monitoring protocol implementation plan for the Sonoran Desert Network.
Ivan, J. S., and E. S. Newkirk. 2016. CPWPhoto Warehouse: a custom database to facilitatearchiving, identifying, summarizing and managing photodata collected from camera traps. Methods in Ecology and Evolution 7:499–504.
Kays, R., B. S. Arbogast, M. Baker‐Whatton, C. Beirne, H. M. Boone, M. Bowler, S. F. Burneo, M. V. Cove, P. Ding, S. Espinosa, A. L. S. Gonçalves, C. P. Hansen, P. A. Jansen, J. M. Kolowski, T. W. Knowles, M. G. M. Lima, J. Millspaugh, W. J. McShea, K. Pacifici, A. W. Parsons, B. S. Pease, F. Rovero, F. Santos, S. G. Schuttler, D. Sheil, X. Si, M. Snider, and W. R. Spironello. 2020. An empirical evaluation of camera trap study design: How many, how long and when? Methods in Ecology and Evolution 11:700–713.
MacKenzie, D. I., J. D. Nichols, G. B. Lachman, S. Droege, J. Andrew Royle, and C. A. Langtimm. 2002. Estimating site occupancy rates when detection probabilities are less than one. Ecology 83:2248–2255.
MacKenzie, D. I., J. D. Nichols, J. Royle, K. Pollock, L. Bailey, and J. Hines. 2006. Occupancy estimation and modeling: Inferring patterns and dynamics of species occurrence. Boston, Ma.: Elsevier.
——. 2018. Occupancy estimation and modeling: inferring patterns and dynamics of species occurrence. Second edition. London, UK: Elsevier.
Parmesan, C., and G. Yohe. 2003. A globally coherent fingerprint of climate change impacts across natural systems. Nature 421:37–42.
Post, E., J. Brodie, M. Hebblewhite, A. D. Anders, J. A. K. Maier, and C. C. Wilmers. 2009. Global population dynamics and hot spots of response to climate change. Bioscience 59:489–497.
Ramsey, F. L., and D. W. Schafer. 2013. The statistical sleuth: A course in methods of data analysis. Third edition. Boston, Ma.: Brooks/Cole, Cengage Learning.
Steenweg, R., J. Whittington, and M. Hebblewhite. 2015. Canadian Rockies Remote Camera Multi-Species Occupancy Project: Examining trends in carnivore populations and their prey using remote cameras in Banff, Jasper, Kootenay, Yoho and Waterton Lakes National Parks. Final Report. University of Montana. 88p.
Steidl, R. J. 2006. Model selection, hypothesis testing, and risks of condemning analytical tools. Journal of Wildlife Management 70:1497–1498.
——. 2007. Limits of data analysis in scientific inference: Reply to Sleep et al., Journal of Wildlife Management 71:2122.
Theobald, D. M., D. L. Stevens, D. White, N. S. Urquhart, A. R. Olsen, and J. B. Norman. 2007. Using GIS to generate spatially balanced random survey designs for natural resource applications. Environmental Management 40:134–146.
Thomas, C. D., A. Cameron, R. E. Green, M. Bakkenes, L. J. Beaumont, Y. C. Collingham, B. F. N. Erasmus, M. F. de Siqueira, A. Grainger, L. Hannah, L. Hughes, B. Huntley, A. S. van Jaarsveld, G. F. Midgley, L. Miles, M. A. Ortega-Huerta, A. T. Peterson, O. L. Phillips, and S. E. Williams. 2004. Extinction risk from climate change. Nature 427:145–148.
Information prepared by Elise Dillingham and Alex Buckisch, Sonoran Desert Network.