Last updated: April 9, 2021
Article
Short-term Forecasting of Vegetation Condition: Potential Management Uses
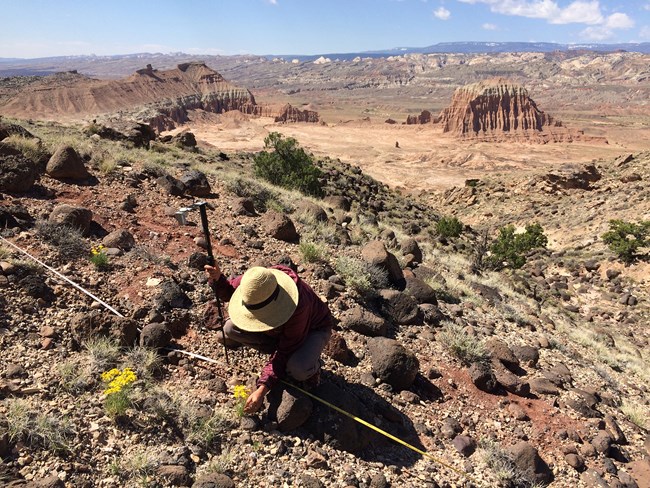
The Issue
On the Northern Colorado Plateau, many park managers have access to data on weather and long-term vegetation change. What they don’t always have is information on how climate affects vegetation from month to month and year to year. Traditional monitoring—which typically involves visiting vegetation plots just once each year—can help answer questions about long-term change and spatial patterns in composition and diversity, but is generally too infrequent for understanding dynamic, within-year response to wet and dry periods. If managers had access to information that could help them to see even a short distance into the future, then short-term decision making could be made easier and more effective.
The Study
A recently completed grassland study demonstrates how monthly satellite measurements of large landscapes, together with climate data and predictive modeling, can help fill in some of these information gaps. An interagency group of scientists, including staff from the Northern Colorado Plateau Network and U.S. Geological Survey, has developed a way to forecast vegetation response to recent weather events at landscape scales on the Colorado Plateau.
The study connects three elements: annual, plot-level species data collected on the ground; high-frequency measurements of landscape greenness from NASA satellites; and associated weather data. The result is a quantitative model that is able to forecast short-term vegetation condition—that is, it has been shown to reliably predict what vegetation condition will be a few months into the future.
Many studies have shown that there is a strong vegetation response to precipitation on an annual or seasonal scale in semi-arid environments. Fewer studies have investigated these relationships at more frequent intervals, such as monthly. Even fewer have included multiple climate and environmental factors that influence vegetation at different times. This study analyzed multiple variables to determine which are most important at different months throughout the year—and within that context, which are the best predictors of vegetation condition later in the growing season.
Findings
The model is based in the understanding that vegetation response lags weather, so plant response to precipitation might come a day, a week, or even months after the precipitation falls. Also, precipitation is not the only environmental factor that affects the availability of water to plants. Measures such as soil moisture and evapotranspiration, called water balance metrics, can be more useful than precipitation alone for examining plant response. In semi-arid environments, high annual, seasonal, and monthly variations in plant productivity can be largely explained by relating climate and water balance factors across time. For instance, on one grassland, soil moisture conditions beginning in February were found to be useful for predicting vegetation abundance in October.
The model was tested using two large grassland sites (1 grazed, 1 ungrazed) at Capitol Reef National Park. In both grasslands, green-up (as observed through the satellite-derived Normalized Difference Vegetation Index, or NDVI), occurred in March through May, in response to soil moisture that had accumulated during the winter. In years without summer monsoon rains, NDVI typically peaked in June. In years with a summer monsoon, NDVI peaked later, generally between August and October.
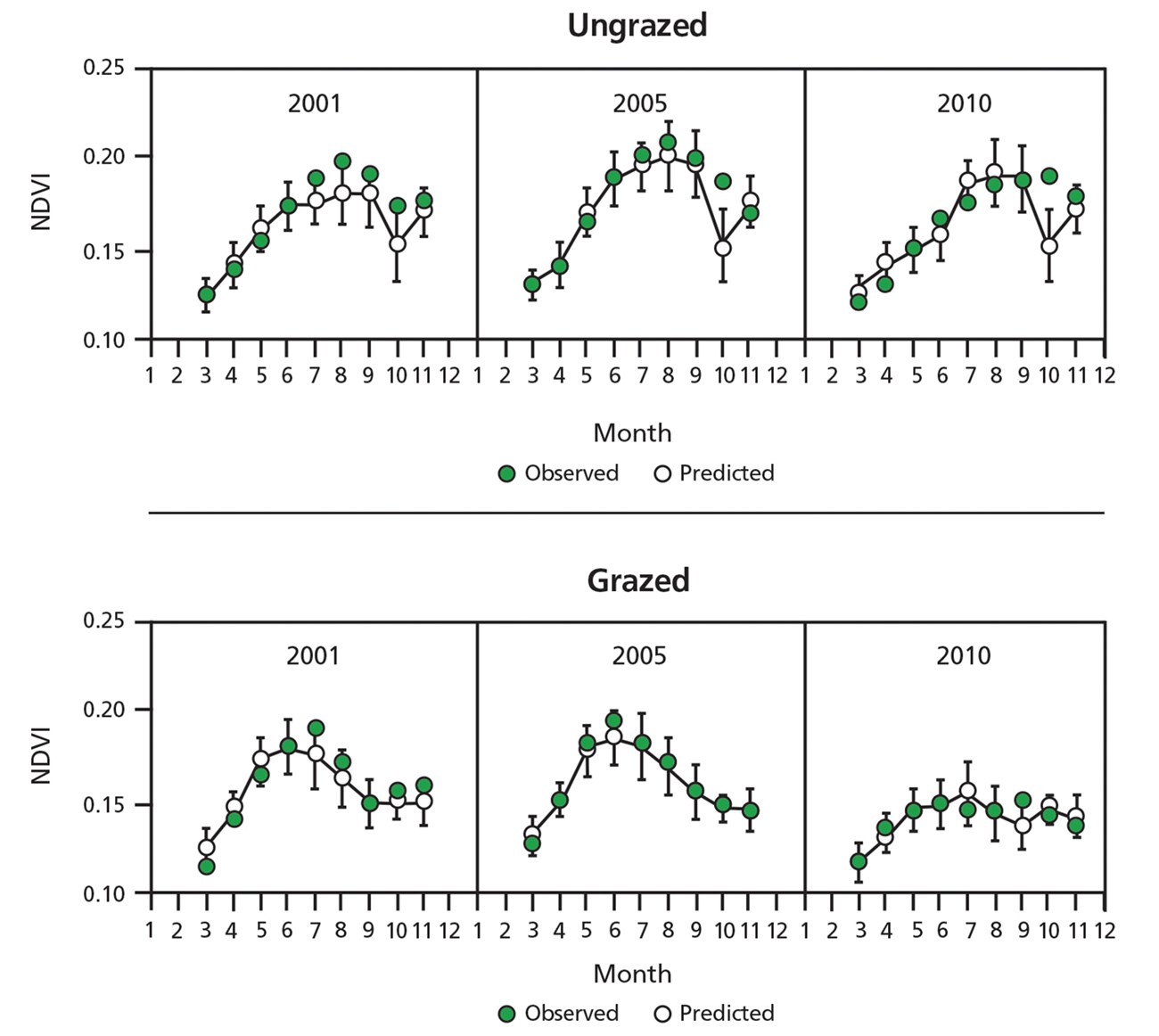
Although the two grasslands responded to climatic conditions similarly, a lack of perfect agreement also suggests differences. For instance, the grazed grassland showed a response to drying conditions one month sooner than the ungrazed grassland. Differences in vegetation composition and abundance help to explain these and other patterns. The ungrazed grassland was more productive throughout the year and also had more vegetation cover and species richness. These results suggest that removal of aboveground biomass or other changes to vegetation structure caused by grazing can affect vegetation response to climate.
The study also found that the ability of vegetation to grow in one year is partly related to what happened during the previous year. If a dry year precedes a wet year, then the reduced biomass levels caused by the dry year limit the potential for vegetation to respond to good conditions in the wet year that follows.
Management Uses
Identifying different climate and water balance windows to explain vegetation greenness can provide park managers with predictions to help inform decisionmaking. If strong, site-specific relationships between the various climate and environmental factors (including land use, vegetation assemblage, and soil type) can be developed at management scales, then it should be possible to make near-term forecasts of vegetation condition simply by tracking weather and water balance.
At parks with grazing, predictive models of vegetation greenness with up to four months of lead time could help managers to set stocking rates that are protective of park resources. Park managers could also use this model, along with other information, to help predict the months and years in which projects that depend on good growing conditions, such as restoration activities, might be most likely to succeed. The model may also help park managers by predicting the short-term impacts of drought on vegetation. Ultimately, this study adds a broad-scale perspective, at high temporal frequency, to the suite of information available for decisionmaking and may expand the ability of managers to apply science to management questions in a timely, cost-effective manner.
For more information, see Thoma, D. P., S. M. Munson, K. M. Irvine, D. L. Witwicki, and E. L. Bunting. 2016. Semi-arid vegetation response to antecedent climate and water balance windows. Applied Vegetation Science 19(3):413–429 or contact David Thoma.