Last updated: February 23, 2021
Article
Improving Methods to Count Wildlife
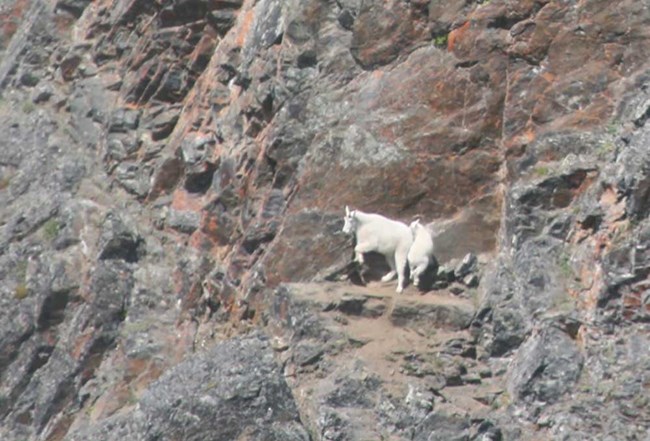
Having accurate counts or estimates of wildlife populations is important for a number of reasons. Monitoring wildlife numbers over time can tell us if the population is thriving or in trouble, we can see patterns in population cycles or correlations between population numbers and harmful events (such as extreme weather). The number of hunting or harvest permits is also based on understanding population dynamics from monitoring population numbers.
We want to improve both the accuracy and efficiency in monitoring wildlife. With the goal of improving estimates of the number of animals on the landscape, we developed a method formally linking two common sampling approaches: large-scale distance sampling surveys and traditional minimum count surveys. To demonstrate the utility of our approach, we combined data from a distance sampling survey of mountain goats across the Kenai Peninsula with minimum counts conducted within individual hunt units. Our results showed that the new approach did, indeed, improve estimates at both the local (i.e., hunt unit) and landscape (i.e., Kenai Peninsula) scales. We expect our work will be useful for joining older minimum count datasets with those collected using newer methods, such as distance sampling, thereby increasing both data quality and continuity through time.
Integrating distance sampling with minimum counts to improve monitoring
Abstract
Minimum counts are commonly used to estimate population size and trend for wildlife conservation and management; however, the scope of inference based on such data is limited by untestable assumptions regarding the detection process. Alternative approaches, such as distance sampling, occupancy surveys, and repeated counts, can be employed to produce detection‐corrected estimates of population parameters. Unfortunately, these approaches can be more complicated and costly to implement, potentially limiting their use. We explored a conceptual framework linking datasets collected at different spatial scales under different survey designs, with the goal of improving inference. Specifically, we link landscape‐scale distance sampling surveys with local‐scale minimum counts in an integrated modeling framework to estimate mountain goat (Oreamnos americanus) abundance at both the local and regional scale in south‐central Alaska, USA, and provide an estimate of detection probability (i.e., sightability) for the minimum counts. Estimated sightability for the minimum count surveys was 0.67 (95% credible interval [CrI] = 0.52–0.83) and abundance for the entire area was 5,600 goats (CV = 9%), both in broad agreement with estimates from previous studies. Abundance estimates at the local scale (i.e., individual min. count unit) were reasonably precise ( = 18%), suggesting the integrated approach can increase the amount of information produced at both spatial scales by linking minimum count approaches with more rigorous survey designs. We propose that our integrated approach may be implemented in the context of a modified split‐panel monitoring design by altering survey protocols to include frequent minimum counts within local count units and intermittent but more rigorous survey designs with inference to the entire study area or population of interest. Doing so would provide estimates of abundance with appropriate measures of uncertainty at multiple spatial scales, thereby improving inference for population monitoring and management. © 2019 The Wildlife Society.
Schmidt, J. H., J. H. Reynolds, K. L. Rattenbury, L. M. Phillips, K. S. White, D. Schertz, J. M. Morton, and H. S. Kim. 2019. Integrating distance sampling with minimum counts to improve monitoring. Journal of Wildlife Management 83(6): 1454-1465.
Integrating Distance Sampling and Minimum Count Data: A Reply to Becker and Herreman
Becker and Herreman submitted a Comment criticizing multiple aspects of our original work and suggested that the assumptions of distance sampling could not be met for the surveys we conducted. In doing so, they also revealed several misconceptions about our work specifically and the properties of distance sampling in general. In our reply we address their misconceptions and clarify some of the more important concepts related to distance sampling approaches. This is how science works. It is reviewed and open to further questioning and clarification. We check and double check our work and value thoughtful challenges. In the end, we were convinced that our original work was correct.
Schmidt, J. H., J. H. Reynolds, K. S. White, D. T. Schertz, J. M. Morton, and H. S. Kim. 2021. Integrating distance sampling and minimum count data: A reply to Becker and Herreman. The Journal of Wildlife Management