Last updated: July 6, 2021
Article
New Methods to Assess Forage Quality for Moose
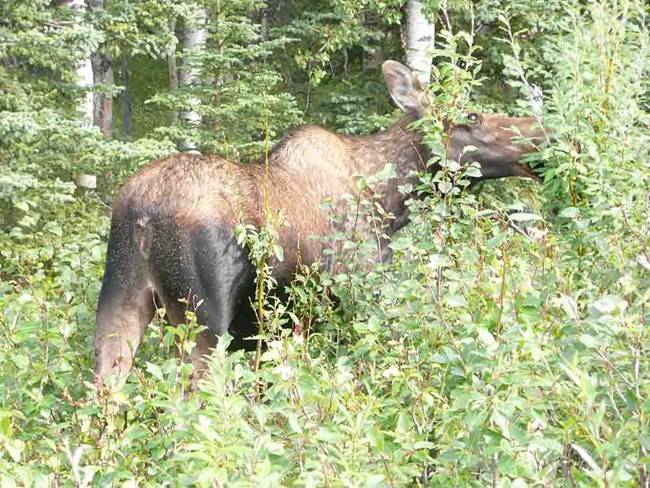
Twenty years ago, ecological studies were often limited by the number of times biologists could find (relocate) their study animals. With the advent and now widespread use of GPS collars, that is no longer a primary concern. Researchers in a 2018 study showed that using more detailed, accurate, and informative habitat information provided stronger, more insightful results than using additional animal relocations.
Animals do not randomly move across the environment. One of the biggest drivers of movement is finding high-quality food sources. High-quality food for ungulates, like moose, is often indexed by how easily its proteins and dry matter are digested. Researchers from the University of Idaho and National Park Service teamed up to show that these measures of forage quality can be determined using specialized cameras mounted in drones. It is hoped that these techniques can be “scaled up” so that key indices of forage quality can be mapped across landscapes and that biologists can be better understand how and why animals utilize their landscape.
Estimating integrated measures of forage quality for herbivores by fusing optical and structural remote sensing data
Abstract
Northern herbivore ranges are expanding in response to a warming climate. Forage quality also influences herbivore distributions, but less is known about the effects of climate change on plant biochemical properties. Remote sensing could enable landscape-scale estimations of forage quality, which is of interest to wildlife managers. Despite the importance of integrated forage quality metrics like digestible protein (DP) and digestible dry matter (DDM), few studies investigate remote sensing approaches to estimate these characteristics. We evaluated how well DP and DDM could be estimated using hyperspectral remote sensing and assessed whether incorporating shrub structural metrics affected by browsing would improve our ability to predict DP and DDM. We collected canopy-level spectra, destructive-vegetation samples, and flew unoccupied aerial vehicles (UAVs) in willow (Salix spp.) dominated areas in north central Alaska in July 2019. We derived vegetation canopy structural metrics from 3D point cloud data obtained from UAV imagery using structure-from-motion photogrammetry. The best performing model for DP included a spectral vegetation index (SVI) that used a red-edge and shortwave infrared band, and shrub height variability (hvar; Nagelkerke R2 = 0.81, root mean square error RMSE = 1.42%, cross validation ρ = 0.88). DDM's best model included a SVI with a blue and a red band, the normalized difference red-edge index, and hvar (adjusted R2 = 0.73, RMSE = 4.16%, cross validation ρ = 0.80). Results from our study demonstrate that integrated forage quality metrics may be successfully quantified using hyperspectral remote sensing data, and that models based on those data may be improved by incorporating additional shrub structural metrics such as height variability. Modern airborne sensor platforms such as Goddard's LiDAR, Hyperspectral & Thermal Imager provide opportunities to fuse data streams from both structural and optical data, which may enhance our ability to estimate and scale important foliar properties.
Jennewein, J. S., J. U. H. Eitel, K. Joly, R. A. Long, A. J. Maguire, L. A. Vierling, and W. Weygint. 2021. Estimating integrated measures of forage quality for herbivores by fusing optical and structural remote sensing data. Environmental Resource Letters 16: 075006. DOI: 10.1088/1748-9326/ac09af