Last updated: August 10, 2022
Article
Improving how we estimate moose abundance
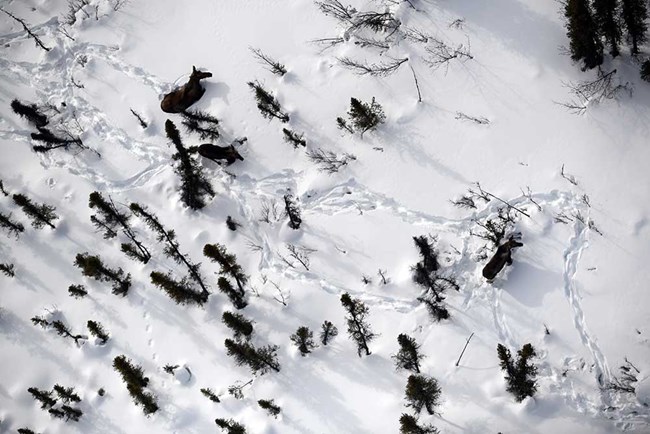
NPS/Matt Cameron
Wildlife management is based on understanding how many individuals exist in a population and whether that number is increasing or decreasing over time and why. However, it is usually not possible to simply count every single individual because animals may be difficult to see and can be spread out across a very large area that is impractical to cover completely. Therefore, most wildlife surveys rely on sampling theory and associated statistical methods that allow us to count a subset of the individuals and then generate a valid estimate of how many occur in the entire area. Given our reliance on sampling, it is important to make sure our estimation techniques are as accurate and efficient as possible. This paper describes a new approach that improves upon the standard method of moose population estimation in use throughout much of Alaska and portions of Canada.
In Alaska and Canada, moose management relies on aerial surveys that are analyzed using what is called the geospatial population estimator (GSPE). The main advantage of the GSPE is that it leverages the spatial autocorrelation among count units (e.g., if one unit has a lot of moose, the one next to it is more likely to have more moose too) to improve estimation. The currently available GSPE software is relatively easy to use and widely employed, but it is relatively inflexible when it comes to incorporating habitat characteristics that influence moose abundance and distribution or including knowledge gained from previous surveys. This in turn limits the types of questions that we can ask of the data (e.g., Is there a trend? What habitat factors affect population size?) and reduces estimator precision. We care about estimator precision because it indicates how confident we are in our estimate of abundance. In order to increase precision (i.e., become more confident in our estimate of abundance), we generally must survey more units. This means that the inherent inflexibilities of the GSPE software could have the effect of making surveys more expensive than would otherwise be necessary.
In this paper, we present a spatial modeling approach that is much more flexible, addresses the above limitations, and provides a robust alternative for analyzing the data from ongoing moose monitoring programs. Using data from six moose surveys in Yukon-Charley Rivers National Preserve conducted between 2003 and 2019, we compared outputs from the GSPE to those based on our new approach. We found that the two approaches produced equivalent estimates of abundance when model structures were the same (i.e., independent surveys, spatial autocorrelation only). However, when we used the new method to consider all six surveys together and included spatial habitat characteristics such as fire history, we were able to establish that the population grew at a rate of 2.3%/year, tracking the increase in previously burned areas within the study area. In addition, the estimator was >40% more precise when using the new approach, which has huge implications for reducing survey cost and effort in the future. Our hope is that the increased flexibility afforded by the new method will help make moose monitoring and management programs more efficient and effective while simultaneously reducing overall cost.
Bayesian spatial modeling of moose count data: Increasing estimator efficiency and exploring ecological hypotheses
Abstract
Moose management throughout much of Alaska and Canada relies on aerial count data, which are commonly collected using the geospatial population estimator (GSPE) protocol. The GSPE uses a model-based analytical approach and finite-population block kriging to estimate abundance from a collection of sampled survey units. Widespread implementation and well-documented analytical software have resulted in reliable estimates of moose abundance, density, and composition across a large proportion of their range. Analysis is conducted almost exclusively using the GSPE software, which fits a fixed model structure to data collected within a single year. The downside of this approach to analysis is that the fixed model structure is inefficient for estimation, leading to more field effort than would otherwise be necessary to achieve a desired level of estimator precision. We developed a more easily modified and flexible Bayesian spatial general additive model approach (BSG) that accommodates spatial and temporal covariates (e.g., habitat characteristics, trend), multiple survey events, prior information, and incomplete detection. Using a series of 6 GSPE surveys conducted in Yukon-Charley Rivers National Preserve, Alaska, USA, from 2003–2019, we established the equivalence of the 2 approaches under similar model structures. We then extended the BSG to demonstrate how a more comprehensive approach to analysis can affect estimator precision and be used to assess ecological relationships. The precision of annual abundance estimators from the BSG were improved by an average of 43% over those based on the standard GSPE analysis, highlighting the very real costs of assuming a fixed (i.e., suboptimal) model structure. The population increased at a rate of 2.3%/year (95% CrI = 0.8–3.8%), and the increase was largely explained by a parallel increase in wildfire extent (i.e., high quality moose habitat). These results suggest that our approach could be used to increase estimator efficiency or decrease future survey costs without any modifications to the basic protocol. While modification of the GSPE software is possible, practitioners may find the BSG approach more convenient for quickly developing model structures for a particular application, thereby allowing them to extract more information from existing and future datasets.
Schmidt, J. H., M. D. Cameron, K. Joly, J. M. Pruszenski, J. H. Reynolds, and M. S. Sorum. 2022. Bayesian spatial modeling of moose count data: Increasing estimator efficiency and exploring ecological hypothesis. The Journal of Wildlife Management 86(5): e22220.