Last updated: January 31, 2022
Article
Connecting large ecological datasets and complex models to improve ecological inference
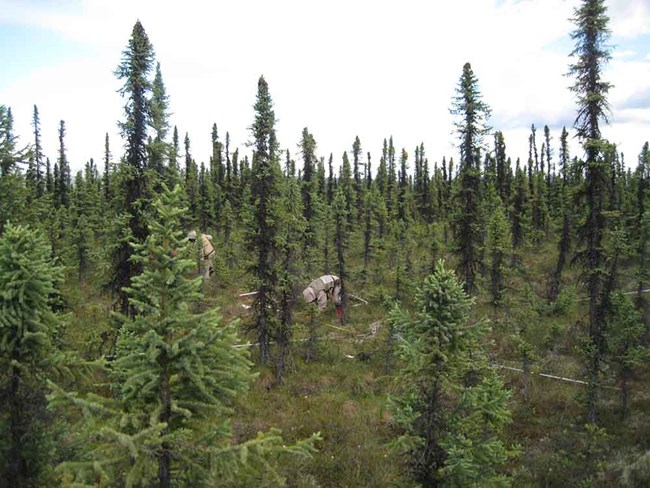
Forests are complex systems that persist over long spans of time. The establishment, growth, and death of individual trees within forests are governed by many factors, not all of which are fully understood (especially over long time spans). The growth of individual trees together, each tree competing for resources and responding to environmental conditions, is a multifaceted web of interactions. When one factor changes, the effects ripple through the forest ecosystem in potentially unexpected ways. As our climate, an important factor regulating tree growth, warms at unprecedented rates in the Far North, scientists are working to understand how these changes will impact forests.
Merging simulation models, robust statistical methods, and ecological field data is a frontier for improving inference and forecasting. Simulation models translate all we know about the factors influencing tree growth over its lifetime—climate, topography, soil conditions, competition—into an interconnected web of equations. The models simulate the patterns of individual trees and then calculate how the aggregation of those individual patterns leads to emergent system properties. Simultaneously, modern statistical techniques, such as machine learning, are rapidly being developed to provide predictions using “big” data in real time. Computational simulation models alone are necessarily incomplete representations of ecosystem functioning, and machine-learning approaches alone may not provide insights into causal mechanisms. Similarly, ecological field data can never fully observe an ecological system. Combining these techniques to understand mechanisms and improve predictions about forest growth dynamics is a promising path forward to advancing ecological understanding.
Bringing these tools together is not always straightforward. In this 2021 paper in Ecology and Evolution, Raiho and others demonstrate how this can be done using pragmatic techniques. They use tree-ring basal area reconstructions in Denali National Park and Preserve to inform successional trajectories of the two most common tree species in the park (black spruce and white spruce) simulated by a forest model, University of Virginia Forest Model Enhanced (UVAFME). The basic principle underlying UVAFME is competition for light, water, and nutrients between individual trees. Changing age, size and number of individual trees then feeds back to the ecosystem states to alter the availability of light, water, and nutrients in the following years. Each year, individual tree growth is constrained by the most limiting of these factors, resulting in realistic forest growth dynamics over successional timescales (hundreds of years).
In addition to demonstrating a pragmatic path forward for improving multi-species representations in vegetation simulation models using long-term field data, the results provide ecological inference about the long-term competitive dynamics between slow-growing black spruce (Picea mariana) and relatively faster-growing white spruce (Picea glauca). The model results show that white spruce may grow faster and black spruce may become growth-stressed sooner than current UVAFME default parameterizations, agreeing with other studies’ assessments (Wirth et al. 2008, Roland et al. 2013, Nicklen et al. 2021) that white spruce may have a competitive advantage over black spruce. The results also point to maximum age as an important factor affecting white spruce growth, contradicting the assumption that early succession is the most vulnerable time for black spruce in competition with white spruce and suggests that understanding which environmental factors lead to long-lived black spruce may help predict future black spruce distribution.
Bridging implementation gaps to connect large ecological datasets and complex models
Abstract
Merging robust statistical methods with complex simulation models is a frontier for improving ecological inference and forecasting. However, bringing these tools together is not always straightforward. Matching data with model output, determining starting conditions, and addressing high dimensionality are some of the complexities that arise when attempting to incorporate ecological field data with mechanistic models directly using sophisticated statistical methods. To illustrate these complexities and pragmatic paths forward, we present an analysis using tree-ring basal area reconstructions in Denali National Park (DNPP) to constrain successional trajectories of two spruce species (Picea mariana and Picea glauca) simulated by a forest gap model, University of Virginia Forest Model Enhanced—UVAFME. Through this process, we provide preliminary ecological inference about the long-term competitive dynamics between slow-growing P. mariana and relatively faster-growing P. glauca. Incorporating tree-ring data into UVAFME allowed us to estimate a bias correction for stand age with improved parameter estimates. We found that higher parameter values for P. mariana minimum growth under stress and P. glauca maximum growth rate were key to improving simulations of coexistence, agreeing with recent research that faster-growing P. glauca may outcompete P. mariana under climate change scenarios. The implementation challenges we highlight are a crucial part of the conversation for how to bring models together with data to improve ecological inference and forecasting.
Raiho, A. M., E. F. Nicklen, A. C. Foster, C. A. Roland, and M. B. Hooten. 2021. Bridging implementation gaps to connect large ecological datasets and complex models. Ecology and Evolution 11(24): 18271-18287.