Part of a series of articles titled Alaska Park Science Volume 20 Issue 1 - Parks as Proving Grounds.
Article
Mapping and Monitoring Landscape Changes Using Structure from Motion from Aircraft
Celia Miller, Michael Hannam, and Deb Kurtz, National Park Service
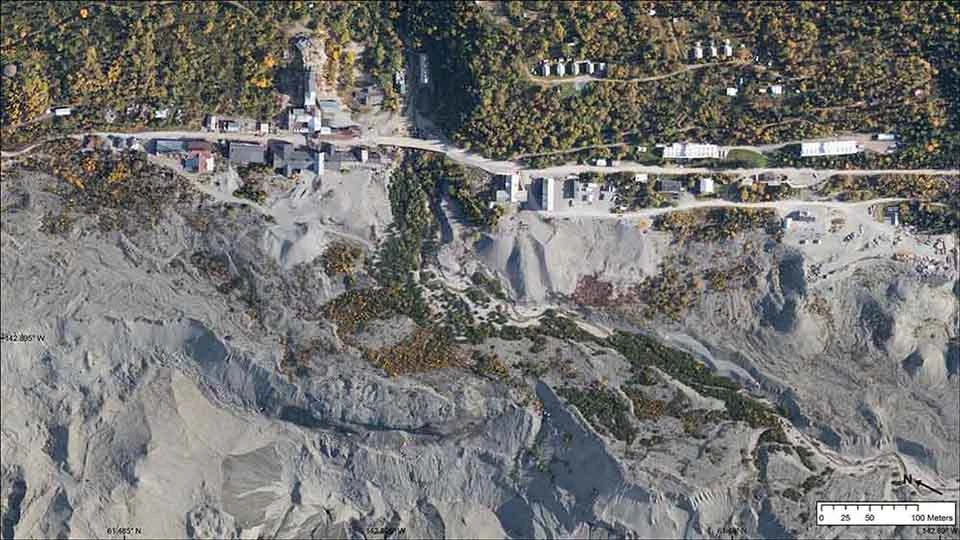
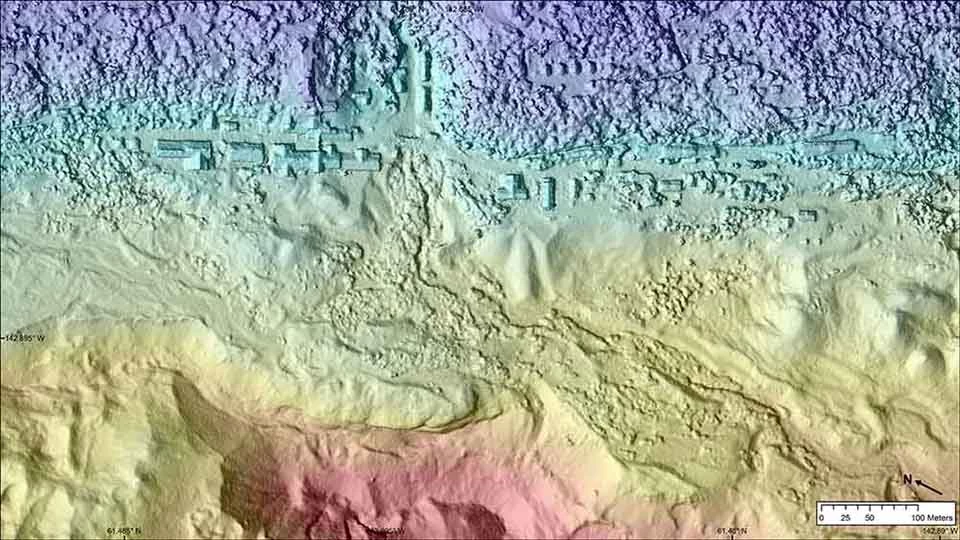
Left image
Orthophoto mosaic of Kennecott mine site.
Right image
Digital elevation map of Kennecott mine site.
To address Alaskan-sized issues in remote parks, remote sensing is often the most useful and efficient tool. Boots-on-the ground collection of biological or physical features is time-consuming and often spatially restricted relative to the vast extent of habitats and size of the physical features of interest. Extrapolations from small sample areas to large areas is often statistically tenuous. As such, remote sensing is useful for monitoring changes on the landscape scale.
Satellite imagery is used for many landscape-scale applications but typically has a maximum resolution of approximately 40 cm. Satellite imagery is useful for parkwide-scale projects (1,000s km2) such as parkwide imagery, a park land cover map, and even detecting elevation changes at larger scales such as in the Arctic digital elevation models. Unmanned Aircraft Systems (UAS) mapping is increasing in use, but UAS flights are commonly restricted to less than 400 feet above ground level and flying line-of-sight, which results in a resolution of about 1-10 cm and constrains them to small-scale projects (<10 km2). They are useful for modeling changes of individual landslides, mapping detailed land cover at a vegetation transect, or making three-dimensional (3D) models of unique landforms (Arches, for example). Aerial photography in aircraft fits in the niche between those two remote sensing systems with typical pixel ground resolutions of 10-60 cm. Aerial structure from motion (SfM) is useful for medium-sized projects (100s km2), such as mapping intertidal environments, detecting landslides over a large area of interest such as an entire park road, and monitoring glacier changes on large glaciers or icefields.
In Alaska, SfM from agency aircraft has provided a cost-effective tool for high-resolution mapping and monitoring of landscape changes to help manage natural resources. Applications so far include monitoring coastal erosion, sea level rise, glacial outburst floods, fires, volcanic eruptions, permafrost thaw, landslides, glacier recession, oil spills, developments, and mining.
Structure from Motion (SFM) Technology
Structure from motion (SfM) technology is the driver behind the recent explosion of unmanned aerial systems (UAS) used for mapping. SfM provides the ability to create high-resolution and accurate orthophoto mosaics and digital elevation models (DEM) using consumer-grade cameras and survey-grade Global Navigation Satellite System (GNSS) equipment. Traditional photogrammetry relied on precisely engineered cameras with known geometry; whereas, SfM technology consists of algorithms that make it possible to use consumer-grade cameras without accurate sensor geometry to generate 3D models. SfM uses multiple overlapping photos to solve for all the variables of a camera geometry, camera orientation and location, and the 3D shape of the object being photographed (Furukawa and Hernandez 2015).
In Alaska, SfM use from aircraft was spearheaded by Dr. Matt Nolan, previously a geophysicist at the University of Alaska Fairbanks (Nolan et al. 2015). The National Park Service (NPS) Alaska Region has worked with Dr. Nolan to apply SfM to various geohazards projects; most notably developing basemap imagery and DEMs for the Denali Park Road and monitoring retrogressive permafrost thaw slumps in Gates of the Arctic National Park and Preserve and Noatak National Preserve (Swanson and Nolan 2018).
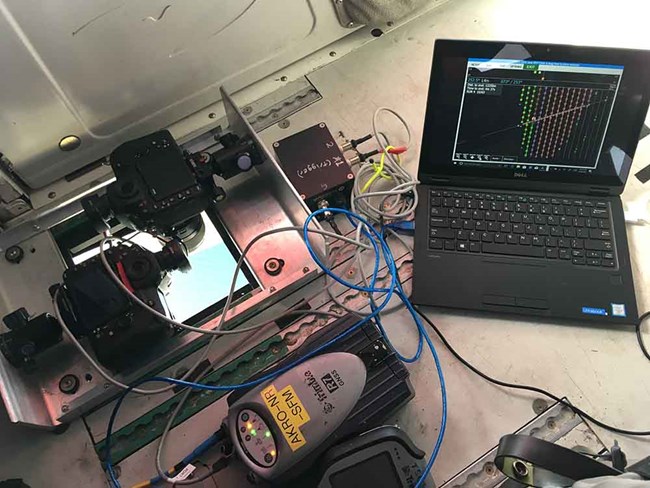
As the pilot follows the flight lines, the cameras are triggered when the plane reaches the camera locations. The shutter flash in the hot-shoe sends an event signal to the running GNSS unit (Trimble™ R7) that records the time of the camera trigger.
With assistance from the U.S. Fish and Wildlife Service and the Bureau of Land Management Cadastral group, the NPS has deployed an aerial SfM system that uses high-resolution digital cameras and survey-grade GNSS units to map and monitor changes using agency planes. The system is shown mounted in a plane in Figure 1 and primarily consists of two digital cameras (standard red-green-blue [RGB], and near infrared [NIR]). The NPS Alaska Regional Office also provides an SfM processing server that can be run remotely by trained park staff.
The NPS Alaska Region is well positioned to deploy aerial SfM because we have numerous agency planes and pilots located in parks near project areas. Area covered in a typical day is about 100–300 km2. For perspective, the city of Anchorage is 250 km2 and can be mapped at a resolution of 15 cm in about 5 hours of flying. If processed just for the DEM and orthophoto mosaic, the project can be completed in less than a week. The primary constraint on the deployment of SfM is weather (Table 1). The Alaska Region is working on outfitting existing NPS planes to be SfM capable and training park employees across the region on the operation of the aerial SfM system. With park planes and personnel trained in acquiring SfM, response times to map and monitor geohazards will be quicker.
2017 | 2018 | 2019 | |
---|---|---|---|
# Days Planned | 14 | 42 | 48 |
# Days Flown | 6 | 23 | 20 |
Success Rate | 43% | 54% | 42% |
# Photos* | 12,315 | 29,996 | 30,106 |
Area Mapped (km2) | 603 | 2,197 | 2,242 |
*Number of photos is doubled when including near infrared (NIR). |
An important caveat is that SfM isn’t useful for measuring ground surface elevations where vegetation is thick, as can be done with LiDAR. Fortunately, many of the parks have high-altitude or high-latitude areas with little to no vegetation. For these places, SfM is as good as LiDAR for elevation mapping (Figure 2; Salach et al. 2018). SfM has the added benefit of producing precise orthorectified imagery, which can be used for vegetation classification.
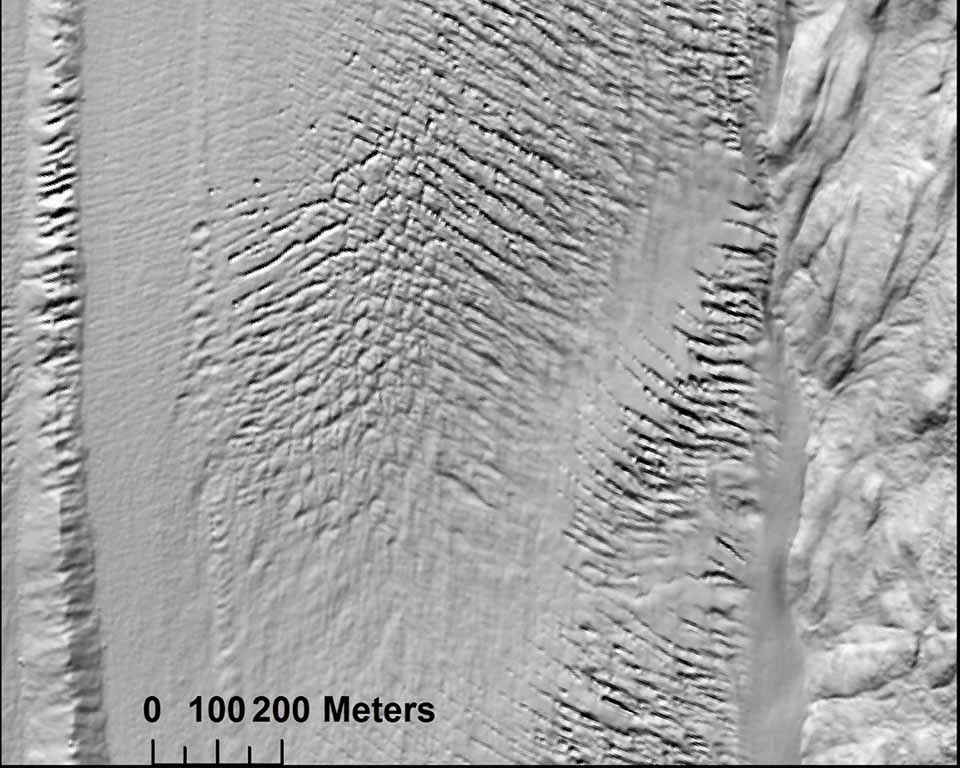
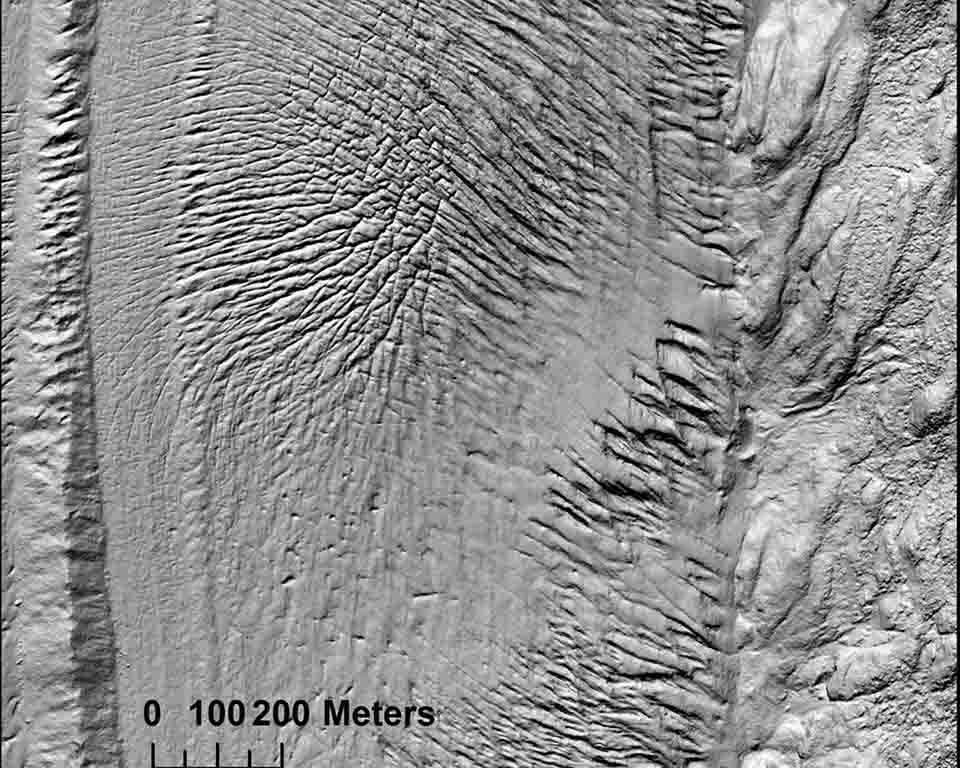
Left image
Digital elevation model of Bear Glacier produced by LiDAR.
Right image
Digital elevation model of Bear Glacier produced by SfM.
Mission Planning and Execution
Missions are developed based on multiple factors: available funds, fuel availability, remoteness, time available, and project purpose. Project purpose dictates the resolution needed, the lighting or seasonal timing constraints, and extent necessary to meet the goals. For example: intertidal mapping projects are planned for the lowest tides of the year, which only last a few hours in a day, whereas land cover classification projects require peak growing season and consistent lighting throughout the acquisition. Project purpose also dictates the accuracy and accuracy validity needed. Ground control points (GCPs) are placed when projects require greater vertical accuracy and validity of the vertical and horizontal accuracy. As such, aircraft landing locations within the area of interest (AOI) are necessary if GCPs are to be placed using the fixed-wing aircraft used to fly SfM, or GCPs and vertical check points can be measured before or after an acquisition by other means.
Flight planning software is used to develop gridded camera locations (shutter events) in order to assure that the photo spacing is optimal for SfM processing. The desired resolution, camera type, and lens are entered into the flight planning software with an outline of the AOI from which a gridded flight plan is generated at the necessary altitude. A user chooses the flight direction (e.g., east–west) to reduce the overall flight time or to follow the orientation of the adjacent terrain for safety. An alternative intervalometer method is used in situations where the object to be mapped is narrow and sinuous like roads, trails, rivers, and shorelines. For this method, we use a synchronized remote shutter control for the two cameras that has a time interval function. When properly executed, both methods create extremely accurate orthophoto mosaics and DEMs.
Processing Protocols and Accuracy Assessments
To create 3D models of landscape features, or digital elevation models (DEM), precise camera shutter event locations or visible GCPs are necessary to reference the 3D model to real-world coordinates. Most UAS work relies on ground control marks to georeference the model, because most don’t have a survey-grade GNSS unit onboard. The Alaska Region aerial SfM system relies on precise (to the scale of centimeters) locations of the camera positions to accurately georeference the imaged landscape. We use GNSS base station (Trimble™ R10) that is ideally located less than 50 km from the project area. Using a local base station to correct the aerial GNSS unit (Trimble™ R7) measurements using the post-processing kinematic (PPK) technique, results in GNSS positions accurate to within 5 cm (90%) resulting in a camera position accuracy of approximately 15 cm. SfM processing is completed in Metashape™ software without using GCPs to inform the model. Survey-grade GNSS measurements on visible GCPs are used to test the horizontal accuracy of the resulting orthophoto mosaics, which show they are accurate to ±15 cm (95%). GCPs and/or vertical check points placed on flat, open bare ground are used to adjust DEMs. After testing accuracy of numerous projects, the DEMs have a precision of about ±10 cm (95%), but all DEMs have a vertical shift of approximately -25 to -70 cm (DEM minus GCP elevations; see Alidoost and Arefi 2017). As such, when vertical accuracy is critical for the project, GCPs or vertical check points are used to adjust the DEMs to account for the vertical shift. With the low vertical error, repeat SfM acquisitions over the same AOI can detect changes of about 20 cm or greater.
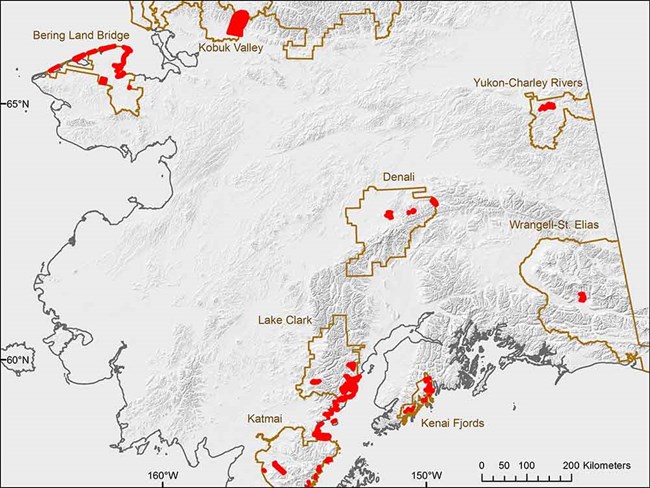
Example Applications
Figure 3 shows the areas that the Alaska Region aerial SfM system has been deployed from 2017 through 2020. Although the system was developed for natural resource projects, it has been used for a wide-range of applications, including:
- coastal ecological and geomorphic mapping;
- intertidal habitat mapping and salt marsh landcover change detection;
- coastal erosion monitoring;
- developing landform maps for archeological sites;
- monitoring landslides;
- monitoring mining;
- mapping abandoned mineral lands;
- mapping rivers for planning stream restoration;
- mapping park infrastructure developments;
- monitoring landcover and permafrost changes after fires;
- monitoring glacier retreat; and
- responding to glacier outburst floods.
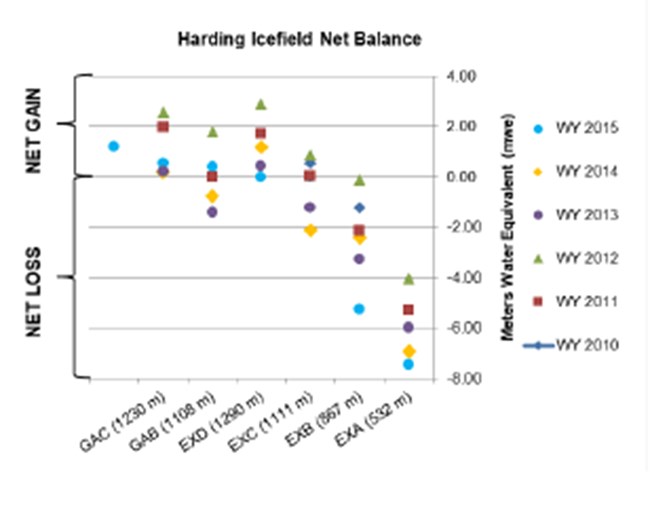
Kenai Fjords National Park Glacier Change Monitoring
Exit Glacier in Kenai Fjords National Park is one of the most visited and easily accessible land-terminating glaciers in North America, yet it is becoming less accessible. Until very recently, the toe of the glacier was easily accessible with a short walk along a flat riverbed. Today, the toe is not accessible because it is constrained in a cliff-sided gorge. As the glacier recedes up the mountainside, the interest for viewing the glacier is receding with it (Moser 2016). SfM is being used by park managers to help monitor and project the rate of retreat in order to make informed decisions about maintaining the infrastructure and managing use in the Exit Glacier area.
Beginning in 2016, SfM has been deployed annually to measure the volume changes for Exit Glacier. Figure 4 shows the vertical change between the 2018 SfM DEM and a 2008 LiDAR-derived DEM. The higher elevation Harding Icefield area has dropped 10–20 meters vertically; whereas, 100 meters of ice has melted from the toe of Exit Glacier. The vertical change shows a net loss of 12.6 m of glacier ice, which equates to an annual average loss of 1.26 m of ice per year. The annual water equivalent gains or losses at the index sites in Figure 5 show that the highest-elevation sites have a slight gain to no change in accumulation and the lower-elevation sites have a net loss. Assuming glacier ice density of 0.9 kg/l, the mean annual meters water equivalent is 1.13 m per year. These data show that the loss of ice at higher elevation, detected using SfM, indicates that the Harding Icefield is losing mass from outlet glaciers faster than it is receiving snow in the accumulation zone, which is leading to the recession of Exit Glacier and all other outlet glaciers.
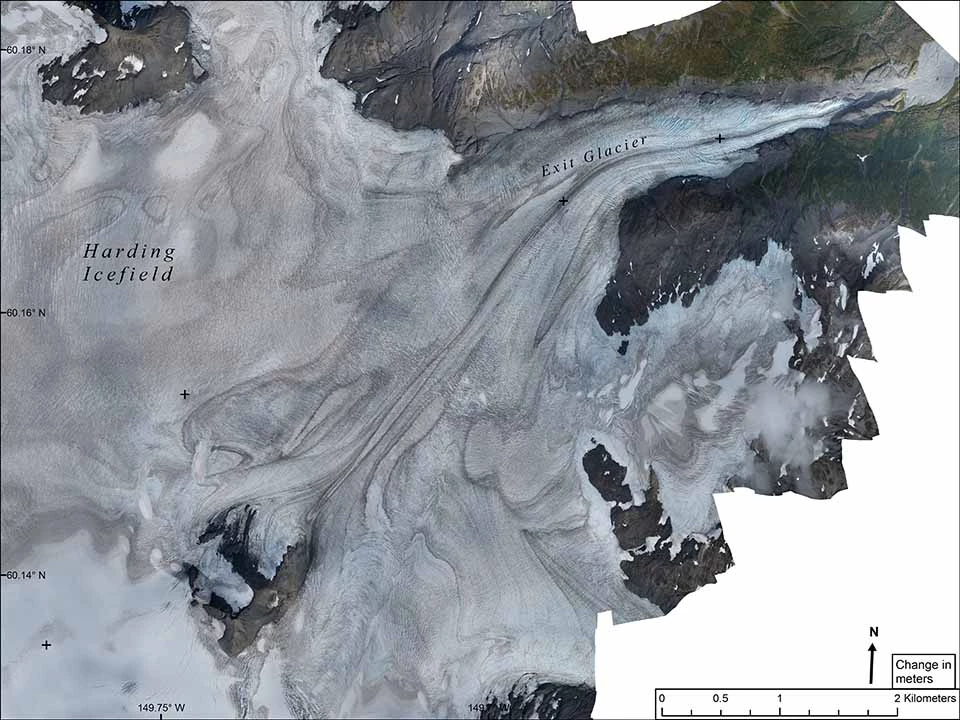
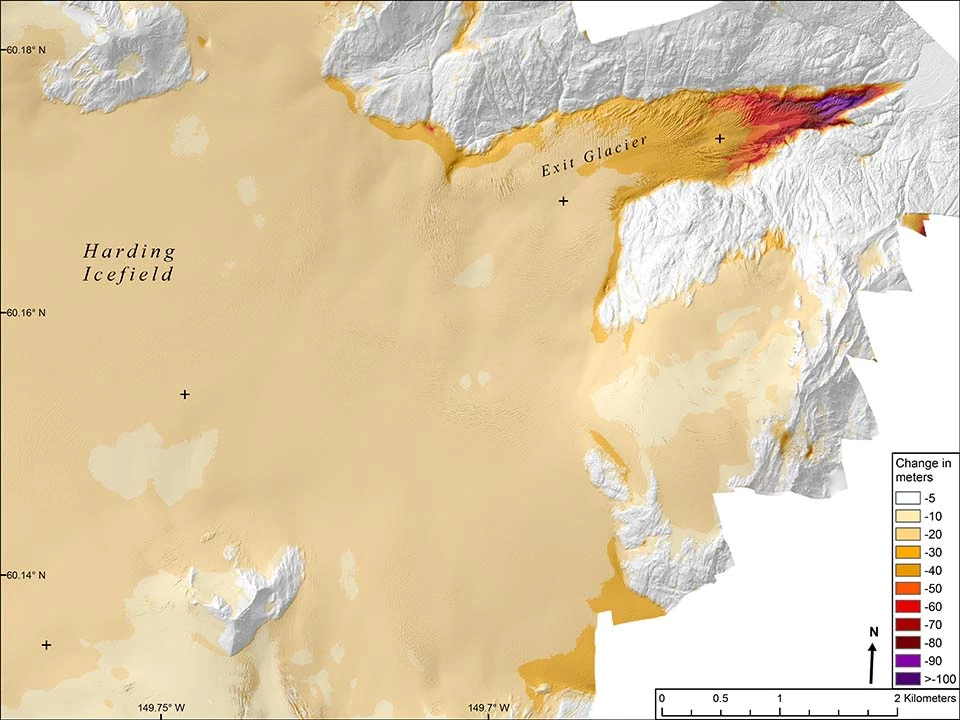
Left image
Harding Icefield orthomosaic, September 2018.
Right image
DEM from the 2008 LiDAR subtracted from the 2018 SfM DEM to show vertical change of the Harding Icefield and Exit Glacier over the 10-year period.
Preparing for Oil Spills through Ecological Classification and Geomorphology of the Western Arctic Coasts
Bering Land Bridge National Preserve and Cape Krusenstern National Monument have approx-imately 1,600 km of predominantly soft-sediment Arctic coastlines rich in biological resources. These shorelines include lagoons with richly patterned interiors, estuaries that are key waterbird breeding areas, extensive salt marshes and brackish wetlands, and sediment transport-driven barrier islands and capes. Over the past decade, marine vessel traffic through the Bering Strait has grown exponentially to take advantage of new ice-free, Arctic summer shipping routes. A new deep-water port servicing the Arctic is planned for Nome, and other oil-related infrastructure is expected. Given the proximity of shipping and nascent industrialization to these formerly remote conservation units, the NPS has embarked on an ambitious coastal research and stewardship plan. A project is underway to develop an ecological classification of coastal vegetation based on geomorphologic and vegetation units defined through remote sensing and field work. SfM was identified as an effective tool for high-resolution mapping of geomorphic features and vegetation classification.
Figure 6 shows a short section of the Bering Land Bridge National Preserve coastline that has been mapped using SfM. Difference analysis of elevations from 2004 LiDAR (flown by the National Oceanic and Atmospheric Administration) to the 2018 SfM shows that along this stretch of coast, the dune ridges and shoreline are progressing inland. Notice that the gross shape of the dunes is preserved, but the crests are shifted a few tens of meters to the south-southwest. Work is underway to conduct a difference analysis along the entire preserve coast.
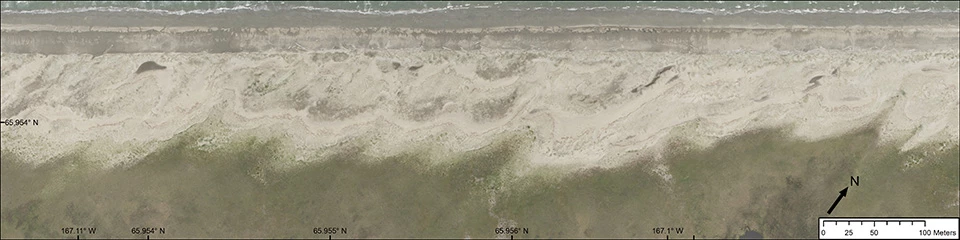
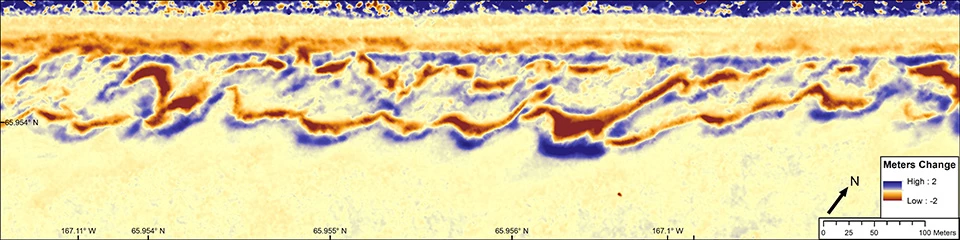
Left image
Orthomosaic of Ikpek Lagoon, Bering Land Bridge National Preserve, 2018.
Right image
Ikpek Lagoon vertical difference layer, the 2018 SfM minus the 2004 LiDAR.
Coastal Landcover Classification
Over the last two years, the Southwest Alaska and Arctic inventory and monitoring networks have partnered with the Alaska Regional Office Natural Resources staff to test using SfM for mapping coastal areas and development of landcover classification routines. Both networks contain abundant pro-ductive coastal habitats that could be impacted by oil spills from oil and gas development or marine vessel traffic.
To map intertidal habitats, we have used both satellite-based and SfM-based landcover classification techniques in order to compare the two resolutions (5 m vs. 20 cm, respectively). Preliminary results for classifying land cover types along the coasts of the two networks are encouraging. Figure 7 shows an example of landcover classification in Bering Land Bridge National Preserve. The combination of RGB and NIR imagery and high-resolution DEM were processed using spectral and object-based classification techniques for land cover types. High-resolution imagery can be tricky for classification because of lighting changes, shadows, and fine details. The example-based classification routine included all four spectral bands (RGB+NIR), NDVI, DEM, topographic position index (TPI), object-based segmentation, and random-forest classification. The imagery mosaics were created using the average of all the 10+ images overlapping every place within the AOI. Using these combinations of layers and averaged mosaics helps reduce the effects of lighting changes throughout the acquisition. It also helps reduce the effects of shadows and the variation in objects when using very high-resolution imagery and increases the contrast between class types. The classes were defined through a multi-year vegetation survey and image interpretation to create hundreds of training points. These tests have proven that SfM can provide a high-resolution tool for quantifying vegetation changes over large areas to better understand and monitor changes to coastal habitat due to tectonic uplift, sea level rise, and climate change in addition to providing a basemap useful for developing oil spill response plans.
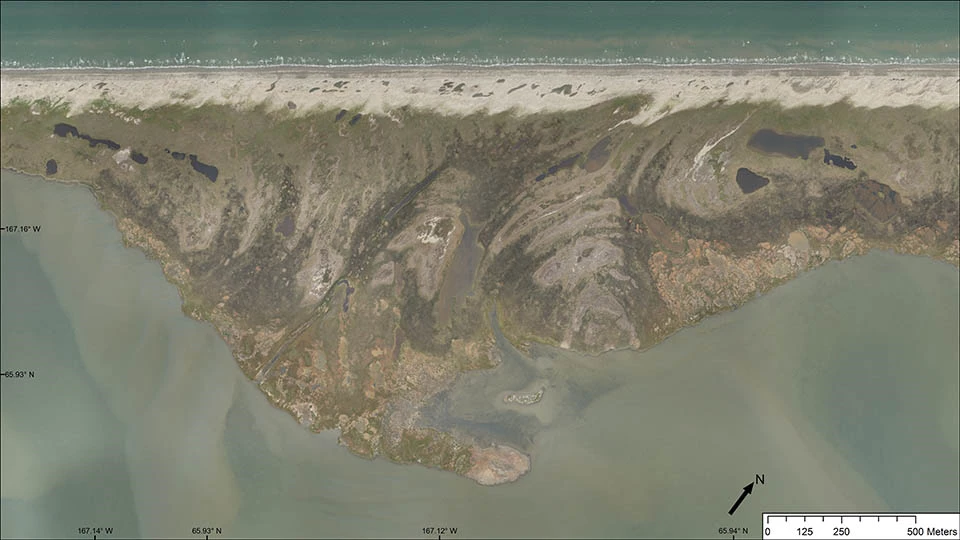
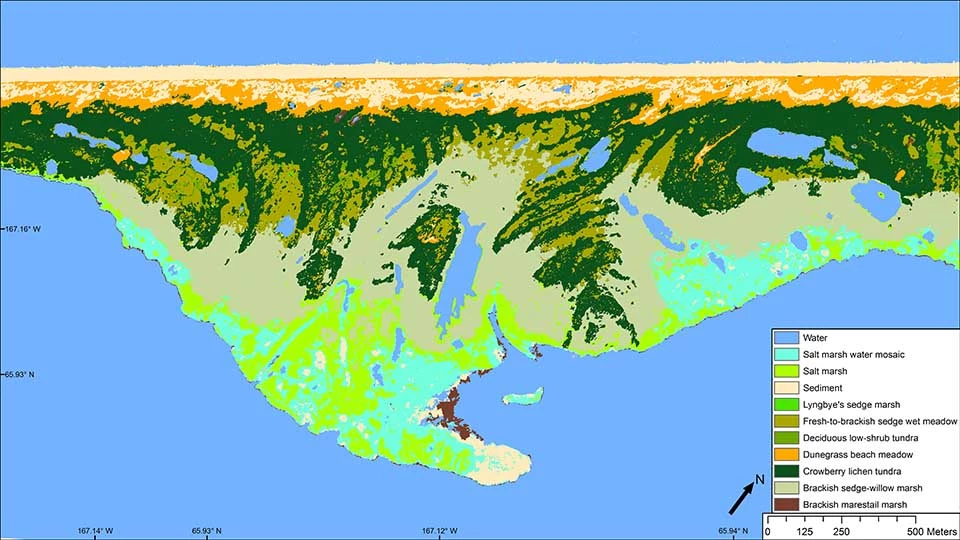
Left image
Orthophoto mosaic of the barrier bar between Ikpek Lagoon and the Bering Sea.
Right image
Landcover classification of the barrier bar between Ikpek Lagoon and the Bering Sea.
Denali National Park and Preserve Landslide Detection Along the Park Road
In 2017, Denali National Park and Preserve had approximately 600,000 visitors, most of whom traveled on tour buses along the Park Road. However, with increased warming, the road is increasingly unstable. As of 2018, the road had about 30 known unstable slopes along the Polychrome section (miles 44 to 46). Recent climate records indicate that the mean annual temperatures are increasing (Sousanes 2016). This anticipated warming is causing permafrost thaw, which is increasing landslide activity in the park (see Capps et al. 2019). Landslides, rockfalls, and debris flows have frequently blocked and damaged the Park Road. The greatest concern is the Pretty Rocks Landslide, which has a displacement rate of approximately 8 cm/day where the road crosses the landslide.
To address the increasing landslide threat to the park road, park management has initiated an un-stable slope management plan and brought numerous tools and partners together to monitor the motion of the landslides (see Capps et al. 2019). Aerial SfM was used in 2018 and 2020 to monitor ground motions in the Polychrome area by flying repeat SfM to detect changes. Figure 8 shows the results of the difference in elevations in 2018, which detected slumping of the road over the Pretty Rocks landslide and intra-slide failures. These vertical changes are only the vertical part of the 3D motion of the landslide; if the landslide moves horizontally, with no change in height, the motion won’t be detected by the DEM differencing. However, the eye can better detect motion of objects; Figure 9 shows an animation made by toggling between the spring and fall 2018 DEMs. In this animation, you can see motion along most of the length of the landslide. Repeating aerial SfM over the greater Polychrome area over the next few years will provide park managers with a tool for detecting landslide motions over the entire area, which will be helpful for making decisions on how to address the road instabilities.
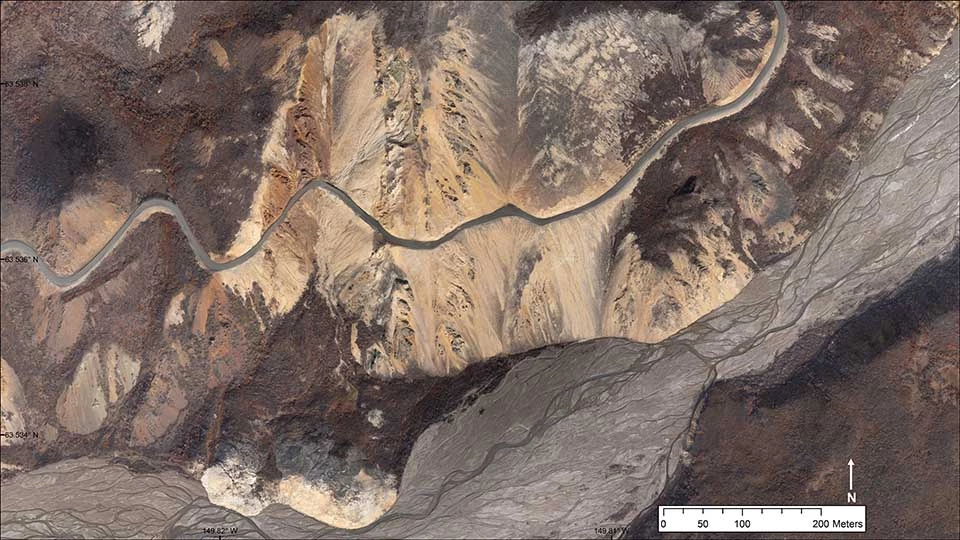
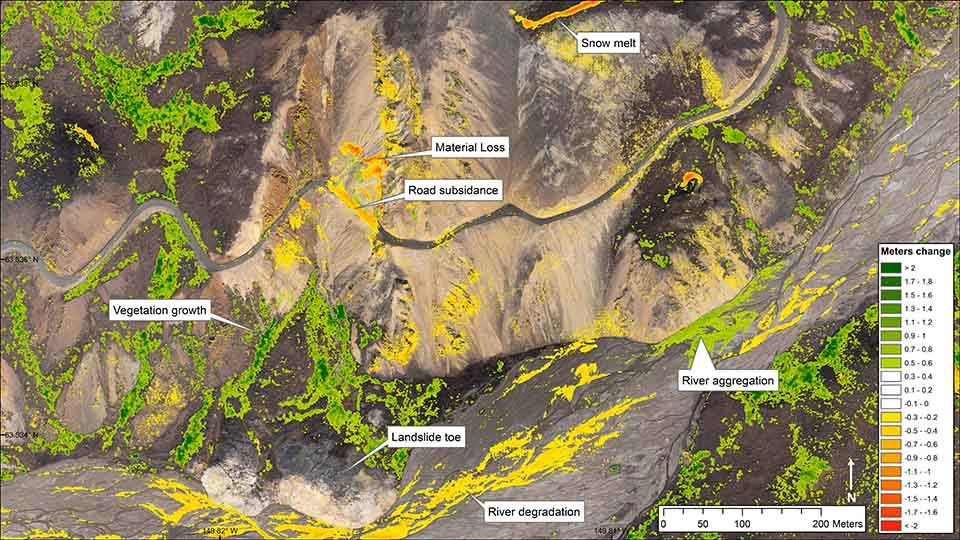
Left image
Aerial image of the Denali Park Road and Polychrome-Pretty Rocks landslide, 2018.
Right image
Denali Park Road and Polychrome-Pretty Rocks landslide DEM vertical difference analysis of SfM between June 6 and September 27, 2018.
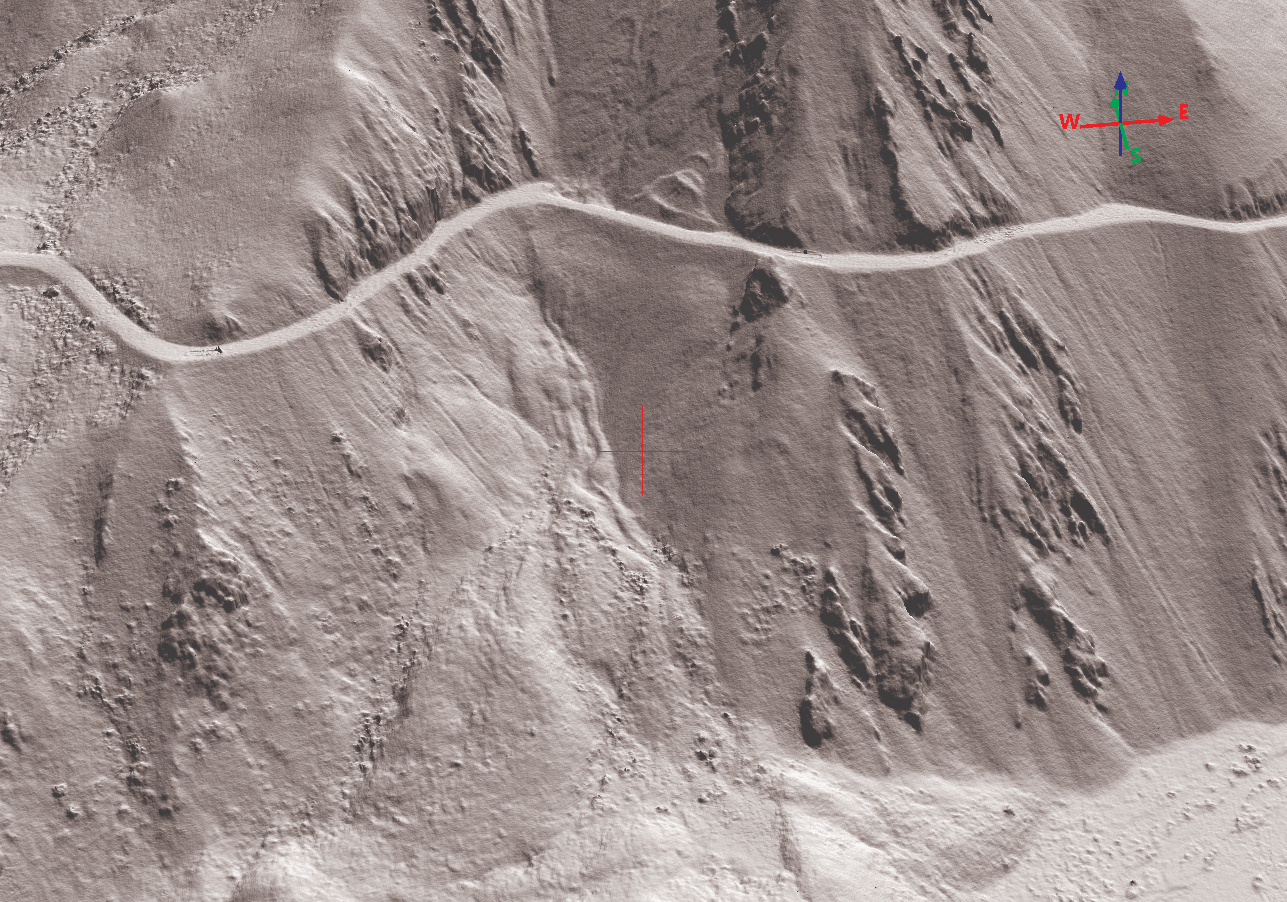
Summary
Aerial SfM is an accessible tool for mapping and monitoring landscape changes for a wide range of applications and disciplines. Using standard consumer-grade cameras and geotagging the images precisely using GNSS hardware is relatively straight forward and most agency pilots have experience flying transects for wildlife surveys. In addition, the rapid growth of SfM using UAS platforms is increasing the number of NPS staff with experience conducting SfM projects. Merging these tools and techniques to develop accurate orthophoto mosaics at the landscape scale is a natural progression. The success of the Alaska Region aerial SfM system during the first four years of testing and deployment has greatly increased the demand for the system. Meeting the growing interest of Alaska park mangers to address the rapidly changing landscapes is the greatest challenge to applying the Alaska Region aerial SfM system to map and monitor Alaska’s dynamic park landscapes.
References
Alidoost, F. and H. Arefi. 2017.
Comparison of UAS-based photogrammetry software for 3D point cloud generation: A survey over a historical site. ISPRS Annals of the Photogrammetry. Remote Sensing and Spatial Information Sciences V-4/W4: 55–61.
Capps, D., D. A. Anderson, and M. McKinley. 2019.
Geohazard risk reduction along the Denali National Park Road. Alaska Park Science 18(1): 44-51.
Furukawa, Y. and C. Hernandez. 2015.
Multi-view stereo: A tutorial. Foundations and Trends in Computer Graphics and Vision 9: 1–148.
Moser, M. C. 2016.
Visitor Responses to the Possible Recession of Exit Glacier at Kenai Fjords National Park. Masters thesis, University of Utah, 81 p.
Nolan, M., C. F. Larsen, and M. Sturm. 2015.
Mapping snow depth from manned aircraft on landscape scales at centimeter resolution using structure-from-motion photogrammetry. The Cryosphere 9: 1445–1463.
Salach, A., K. Bakuła, M. Pilarska, W. Ostrowski, K. Górski, and Z. Kurczynski. 2018.
Accuracy assessment of point clouds from LiDAR and dense image matching acquired using the UAV platform for DTM creation. ISPRS International Journal of Geo-Information 7: 16.
Sousanes, P. 2016.
Denali Climate and Weather Monitoring. Available at https://www.nps.gov/articles/denali-crp-climateweather-monitoring.htm (accessed December 18, 2018)
Swanson, D. K. and M. Nolan. 2018.
Growth of retrogressive thaw slumps in the Noatak Valley, Alaska, 2010-2016, measured by airborne photogrammetry. Remote Sensing 10: 25.
Last updated: May 18, 2021