Last updated: April 5, 2021
Article
Advancements in Analytical Approaches Improve Whitebark Pine Monitoring Results
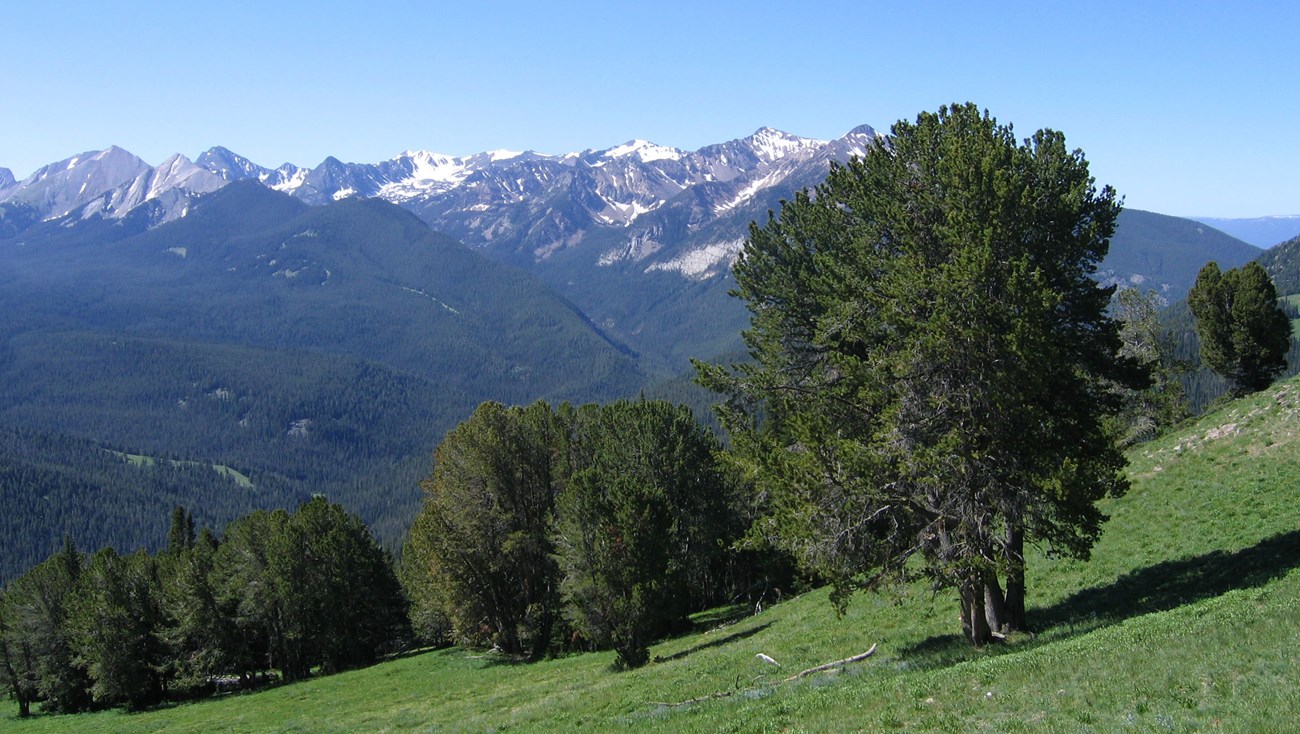
NPS/John Fothergill
Issue
Long-term monitoring programs track the status and trends of species in increasingly vulnerable environments. These monitoring results provide critical information for evaluating, understanding, and managing natural resources. To accurately interpret if and how conditions may be changing for select ecological indicators, it is essential that monitoring programs adopt methods to ensure exceptional data quality. To do this and remain relevant, practitioners need to be flexible and willing to embrace a degree of adaptivity in their protocols. They must periodically re-evaluate their statistical methods and field data collection techniques to provide contemporary, significant, and applicable inferences.
Whitebark pine (Pinus albicaulis) is a declining keystone species of high elevation forests. In 2020, it was proposed for listing as threatened under the Endangered Species Act by the US Fish and Wildlife Service. Scientists have been monitoring the health of whitebark pine in the Greater Yellowstone Ecosystem since 2004. The Interagency Whitebark Pine Monitoring Program is led by the Greater Yellowstone Inventory and Monitoring Network and follows a peer-reviewed protocol. A recent evaluation of the protocol’s analytical methods revealed an underestimation in the prevalence of white pine blister rust (Cronartium ribicola; blister rust), a principle whitebark pine stressor. The study outcomes suggested adjustments to the monitoring protocol. These modifications will improve accuracy by using more contemporary and flexible, model-based approaches and data collection techniques. Maintaining the protocol’s relevancy is especially critical given threats to the species’ survival and the protocol’s use by several other agencies that also track whitebark pine health.
Limits of Field-Based Observational Studies
Long-term monitoring programs that are field-based observational studies present a unique suite of challenges rarely encountered in controlled, laboratory settings. For example, in long-term studies, field crew members change frequently due to the seasonal nature of field work. Environmental conditions, like the weather, are unpredictable. Topography can differ considerably among sites. Study subject attributes can also vary, such as tree height, position in the tree stand, and density of trees for whitebark pine. Individually and collectively, these factors can significantly affect data quality and consistency. While specific adjustments, including extensive training, can help, some issues cannot be fully resolved. In turn, the inherent limitations of field-based observational studies can present substantial statistical challenges.
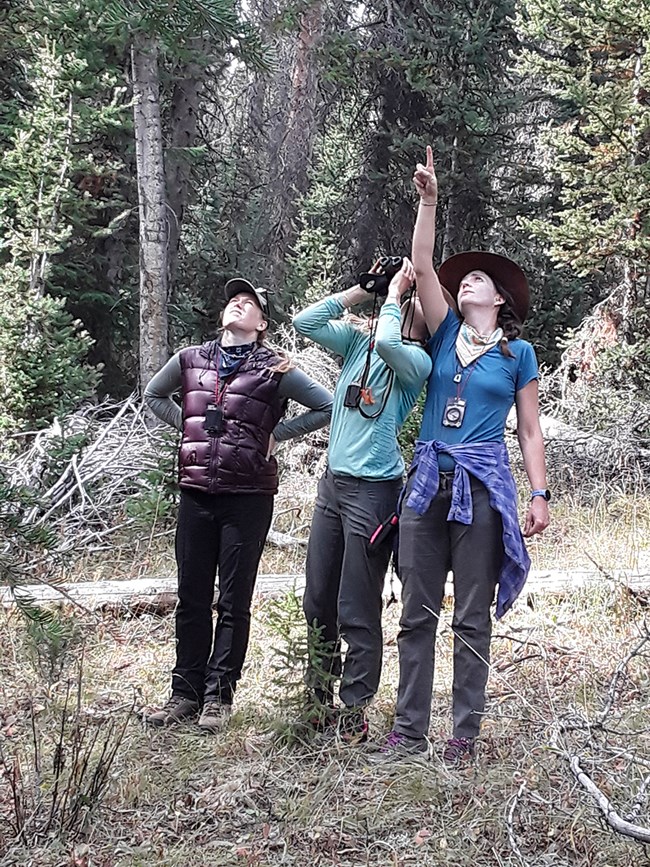
NPS/Erin Shanahan
Imperfect Detection
In field-based studies, a known source of potential bias in statistical estimation is non-detection error. This occurs when observers mistakenly record a species or characteristic of a species as absent (false negative). Observer error, also referred to as imperfect detection, often complicates our ability to estimate populations accurately. Wildlife research has widely recognized and mitigated for imperfect detection by using occupancy models. These techniques explicitly account for imperfect detection. In contrast, vegetation studies rarely incorporate observation and measurement error. They have assumed that detection error is insignificant because plants do not move like animals. However, plant stature, coloration, and positioning among other vegetation are just a few traits that can make detection challenging. Thus, an inherent degree of detection uncertainty often occurs in most vegetation studies. For estimates of status to be credible and useful, study protocols should address imperfect detection when it may impact results.
Imperfect detection can apply to a variety of measurements. In general, “status” refers to the distribution or abundance in a specific time and place of a subject of interest. With longer lived species such as whitebark pine, the distribution and abundance of the tree itself may be less useful than the status of biological threats to the tree. In the context of this investigation, the “status” of blister rust infection in whitebark pine trees was the primary measure. Yet detecting blister rust infection can be challenging in an individual whitebark pine tree or the stand in which the tree grows. Blister rust can be hard to see in the upper branches of tall trees. It can also be difficult to assign to a particular tree if its branches are intertwined with neighboring trees.
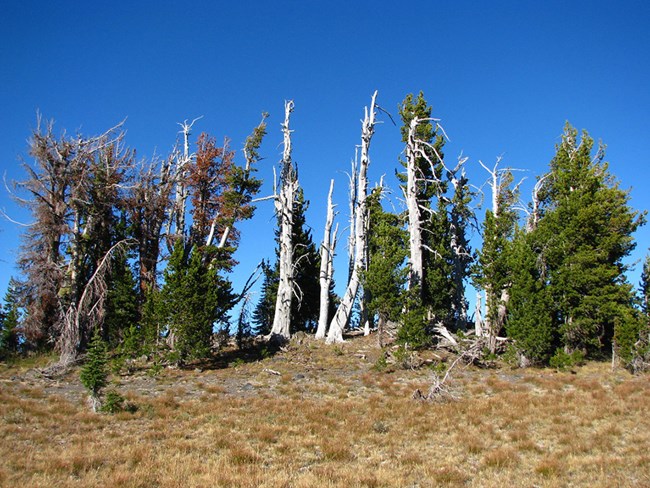
NPS/John Fothergill
Confounding Factors
A second analytical challenge occurs when attempting to account for multiple biotic and abiotic factors that can influence findings. With whitebark pine, for example, another important stressor complicates estimates of blister rust infection prevalence: the mountain pine beetle (Dendroctonus ponderosae). Epidemic levels of this native beetle during an outbreak in the mid-2000s killed 70% of overstory whitebark pine. This shifted the overall population of whitebark pine in the Greater Yellowstone Ecosystem to smaller trees, which have a lower probability of being infected compared to larger trees. This dynamic should be considered in blister rust prevalence assessments. Another factor to address in the whitebark pine monitoring protocol is the variable number of trees in each belt monitoring transect. This variation means that a transect with many trees disproportionately affects infection estimates unless adjustments are made in the analysis.
The Study and Findings
With advances in statistical methodologies, this study explored the most appropriate analytical technique for estimating blister rust infection prevalence in whitebark pine in the Greater Yellowstone Ecosystem. Specifically, we compared the existing protocol’s design-based estimator with a new model-based approach that explicitly accounts for imperfect detection and time and place variation in tree-level blister rust infection probabilities.
To evaluate the monitoring protocol, we zeroed in on one of its key statistics: the prevalence of blister rust infection in whitebark pines. Blister rust infection status in the Greater Yellowstone Ecosystem has been generated using a design-based ratio estimator, which given the study design, was a logical analytical choice when the protocol was initiated (circa 2003). However, a ratio estimator approach limits our ability to investigate the multiple biotic and abiotic nuances that influence blister rust infection and detection throughout the population. It also limits our ability to investigate imperfect detection over the 16 years of this study.
The study revealed shortcomings in the design-based ratio estimator’s ability to accurately assess blister rust prevalence in whitebark pine populations. The ratio estimator did not account for imperfect detection. It also limited the exploration of environmental and biological factors that better explained changes in infection rates over time. As a result, this initial method underestimated the prevalence of blister rust infection in the general whitebark pine population in the Greater Yellowstone Ecosystem (Figure 1). The method also underestimated the probability of a single tree being infected.
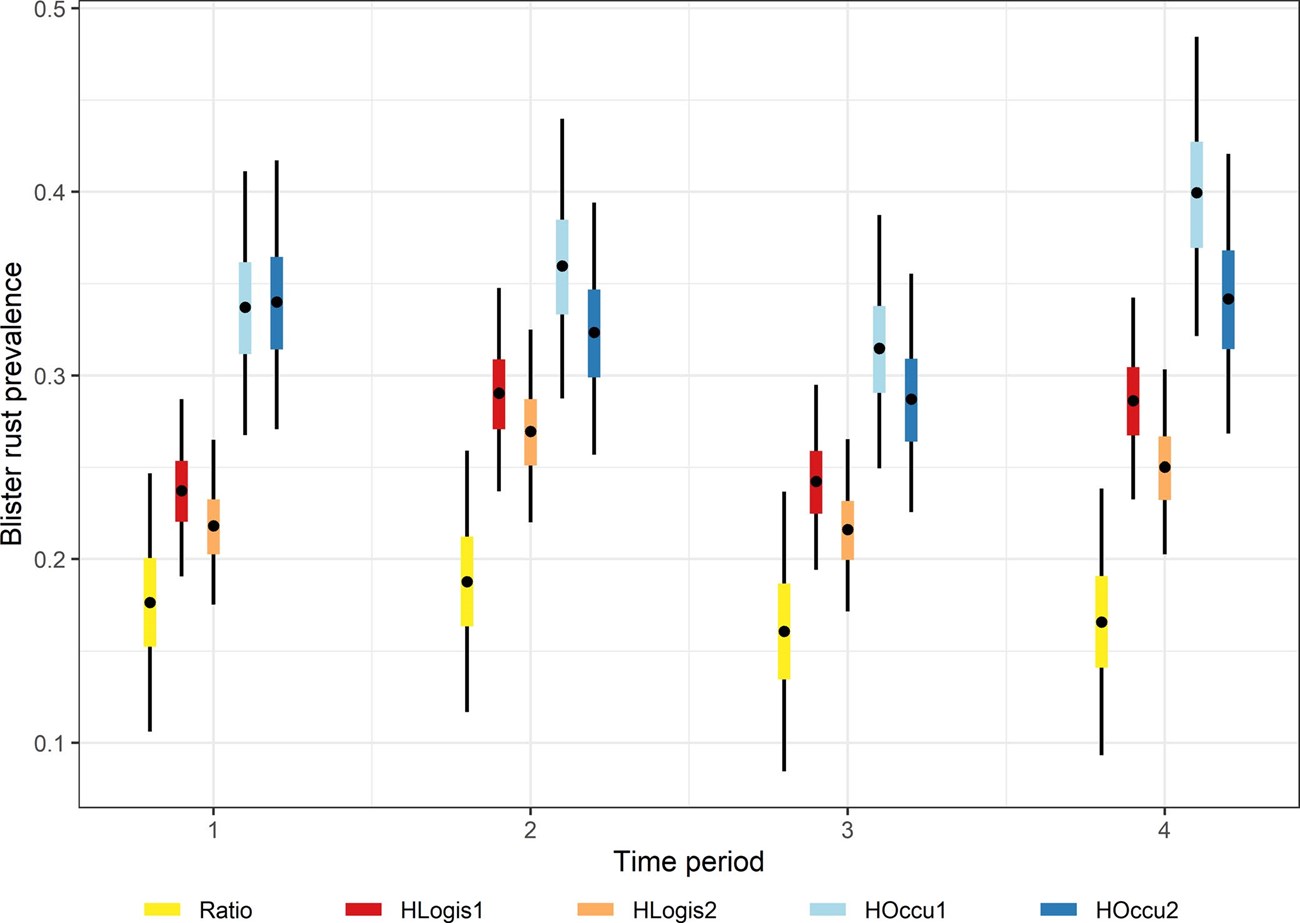
From Shanahan et al. Adaptive monitoring in action: Reconsidering design-based estimators reveals underestimation of whitebark pine disease prevalence in the Greater Yellowstone Ecosystem. J Appl Ecol. 2021;00:1–11.
Figure 1. Estimates of overall blister rust prevalence in whitebark pine trees in the Greater Yellowstone Ecosystem from different analyses. The ‘Ratio’ estimator is the design-based estimator that accounts for the two-stage cluster sampling design but ignores observation errors and heterogeneity in infection probabilities across trees (yellow lines). The ‘HLogis’ is a hierarchical logistic regression model and we fit two versions—one with no covariates (denoted ‘HLogis1’, red lines) and another that included tree DBH and stand elevation to model infection probabilities (denoted ‘HLogis2’, orange lines). The HOccu models account for imperfect detection and we also fit two versions without any covariates (denoted ‘HOccu1’, light blue lines) and with additional infection-level and detection-level covariates (denoted ‘HOccu2’, dark blue lines). Points show the point estimates (posterior means for each model), thick lines show the 50% intervals and thin lines show the 95% intervals.
In comparison, our investigation demonstrated that analysis using a Bayesian hierarchical occupancy model provided several advantages (Figure 2). It offered flexibility in accounting for imperfect detection and inconsistencies in the sampling design. It also accounted for explanatory variables that provide a more comprehensive assessment of changes in blister rust infection over time.
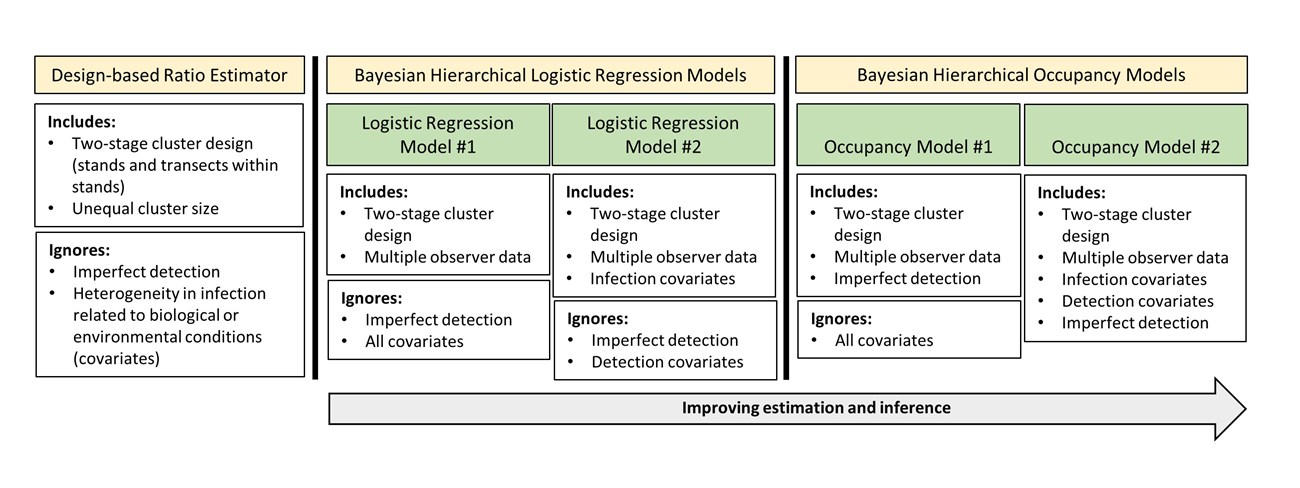
NPS/Erin Shanahan
Figure 2. This study evaluated the original design-based ratio estimator and four Bayesian hierarchical model-based approaches. Moving from left to right, the ratio estimator considers the fewest factors while occupancy model #2 incorporates the most, including both infection and detection covariates. Infection covariates explain heterogeneity in infection probabilities: tree-level DBH (as DBH increases, so does the probability of infection) and stand elevation. Detection covariates explain why observers may have failed to detect infection: tree-DBH (infection in the canopy of larger trees is harder to detect), transect slope, transect hiking time, and observer experience.
Recommendations
Consider Model-Based Approaches
We recommend utilizing more flexible, model-based approaches, such as Bayesian hierarchical models, for status and trend assessments of significant natural resources. Data from observational studies has inherent uncertainty. This uncertainty can be quantified in multiple ways. While our early use of a design-based ratio estimator takes into consideration the protocol structure and design, the model-based approach accounts for the numerous sources of variation inherent in a long-term monitoring study such as ours. The scope of information provided by a model-based analysis is key to informing management and policy decisions for conserving at-risk whitebark pine populations.
Periodically Re-evaluate Protocols
Our appraisal underscores the overarching need to continually review and update a monitoring program’s sampling design and analytical procedures to maintain relevancy. Periodic model assessment and diagnostics may reveal that originally planned statistical analyses can be improved in their ability to adequately describe collected data. As analytical practices advance, we will continue to enhance our estimation expertise. Improved estimates further our understanding of species status and trends gained through long-term monitoring. By periodically re-evaluating our protocol, we are better equipped to estimate status and trends of iconic whitebark pine.
NPS
Management Uses
Long-term ecological monitoring plays a key role in environmental policy and species status decisions. Monitoring is essential in managing natural resources where it can help uncover the mechanisms driving ecological change. In the Greater Yellowstone Ecosystem, blister rust impacts on whitebark pine are influenced by randomly transpiring events such as the recent mountain pine beetle outbreak, other stand-altering occurrences like wildfires, and projected changes in climate. By tracking these interacting impacts with effective analysis tools like Bayesian modeling approaches, we can identify significant patterns of blister rust infection and communicate the potential consequences of this pathogen on future generations of whitebark pine. In this way, long-term monitoring data analyzed with the latest methods helps park managers understand complex relationships. It provides them with contemporary data and enables sound decision making for protecting and restoring whitebark pine on the landscape.
More Information
Read the study
1 Shanahan E, Wright WJ, Irvine KM. Adaptive monitoring in action: Reconsidering design-based estimators reveals underestimation of whitebark pine disease prevalence in the Greater Yellowstone Ecosystem. J Appl Ecol. 2021;00:1–11. https://doi.org/10.1111/1365-2664.13837
Contact
Erin Shanahan, Ecologist
NPS Inventory and Monitoring Division
Greater Yellowstone Network
erin_shanahan@nps.gov
406-994-7530
Kathryn M. Irvine, Research Statistician
US Geological Survey
Northern Rocky Mountain Science Center
kirvine@usgs.gov
Download a pdf of this article